5分钟构建RAG管道
RAG通过提供上下文相关的数据增强大型语言模型的响应。本教程演示了如何使用Qdran和DeepSeek快速构建RAG管道。
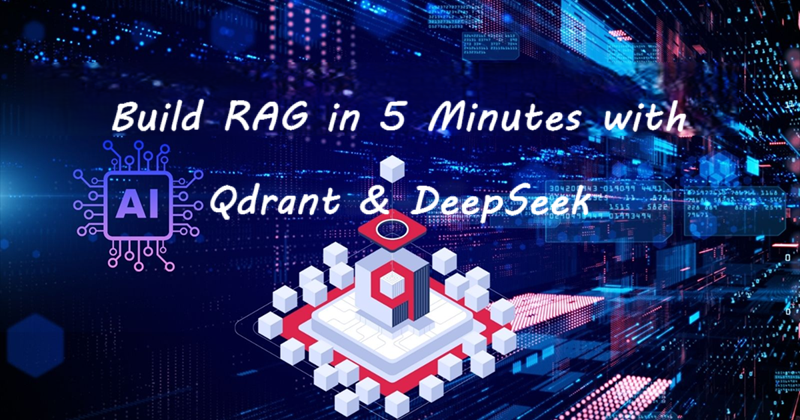
本教程演示了如何使用Qdrant作为向量存储解决方案和DeepSeek进行语义查询增强来构建 检索增强生成(RAG) 管道。RAG管道通过提供上下文相关的数据增强大型语言模型(LLM)的响应。
1、概述
在这个教程中,我们将:
- 使用FastEmbed将样本文本转换为向量。
- 将向量发送到Qdrant集合。
- 将Qdrant和DeepSeek连接成一个最小化的RAG管道。
- 向DeepSeek提出不同的问题并测试答案准确性。
- 使用从Qdrant检索的内容丰富DeepSeek提示。
- 在之前和之后评估答案准确性。
2、架构
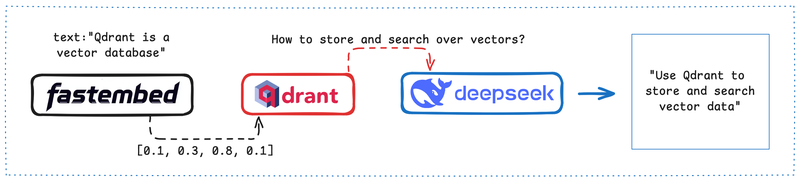
3、先决条件
确保你具备以下内容:
- Python环境(3.7+)
- 访问Qdrant Cloud
- 来自DeepSeek平台的DeepSeek API密钥
4、安装Qdrant
pip install "qdrant-client[fastembed]"
Qdrant将充当知识库,为我们将要发送给LLM的提示提供上下文信息。
你可以在http://cloud.qdrant.io获取一个永久免费的Qdrant云实例。了解如何设置您的实例,请参阅快速入门。
QDRANT_URL = "https://xyz-example.eu-central.aws.cloud.qdrant.io:6333"
QDRANT_API_KEY = "<your-api-key>"
5、实例化Qdrant客户端
import qdrant_client
client = qdrant_client.QdrantClient(url=QDRANT_URL, api_key=QDRANT_API_KEY)
6、构建知识库
Qdrant将使用我们事实的向量嵌入来丰富原始提示的上下文。因此,我们需要存储这些向量嵌入以及用于生成它们的事实。
我们将使用bge-small-en-v1.5模型通过FastEmbed——一种轻量级、快速的Python嵌入生成库。
Qdrant客户端提供了与FastEmbed的便捷集成,使得构建知识库非常简单。
client.set_model("BAAI/bge-base-en-v1.5")
client.add(
collection_name="knowledge-base",
# The collection is automatically created if it doesn't exist.
documents=[
"Qdrant is a vector database & vector similarity search engine. It deploys as an API service providing search for the nearest high-dimensional vectors. With Qdrant, embeddings or neural network encoders can be turned into full-fledged applications for matching, searching, recommending, and much more!",
"Docker helps developers build, share, and run applications anywhere — without tedious environment configuration or management.",
"PyTorch is a machine learning framework based on the Torch library, used for applications such as computer vision and natural language processing.",
"MySQL is an open-source relational database management system (RDBMS). A relational database organizes data into one or more data tables in which data may be related to each other; these relations help structure the data. SQL is a language that programmers use to create, modify and extract data from the relational database, as well as control user access to the database.",
"NGINX is a free, open-source, high-performance HTTP server and reverse proxy, as well as an IMAP/POP3 proxy server. NGINX is known for its high performance, stability, rich feature set, simple configuration, and low resource consumption.",
"FastAPI is a modern, fast (high-performance), web framework for building APIs with Python 3.7+ based on standard Python type hints.",
"SentenceTransformers is a Python framework for state-of-the-art sentence, text and image embeddings. You can use this framework to compute sentence / text embeddings for more than 100 languages. These embeddings can then be compared e.g. with cosine-similarity to find sentences with a similar meaning. This can be useful for semantic textual similar, semantic search, or paraphrase mining.",
"The cron command-line utility is a job scheduler on Unix-like operating systems. Users who set up and maintain software environments use cron to schedule jobs (commands or shell scripts), also known as cron jobs, to run periodically at fixed times, dates, or intervals.",
]
)
7、设置DeepSeek
RAG改变了我们与大型语言模型交互的方式。我们将一个知识导向的任务转换为语言导向的任务,后者期望模型提取有意义的信息并生成答案。当正确实现时,LLM应该执行语言导向的任务。
任务开始于用户发送的原始提示。然后,相同的提示被向量化并用作最相关事实的搜索查询。这些事实与原始提示结合,构建一个包含更多信息的较长提示。
但让我们从直接提问开始。
prompt = """
What tools should I need to use to build a web service using vector embeddings for search?
"""
使用Deepseek API需要提供API密钥。你可以从DeepSeek平台获取它。
现在我们可以最终调用完成API。
import requests
import json
# Provide your own Deepseek API key
# from https://platform.deepseek.com/api_keys
API_KEY = "<YOUR_DEEPSEEK_KEY>"
HEADERS = {
'Authorization': f'Bearer {API_KEY}',
'Content-Type': 'application/json',
}
def query_deepseek(prompt):
data = {
'model': 'deepseek-chat',
'messages': [
{'role': 'user', 'content': prompt}
],
'stream': False
}
response = requests.post("https://api.deepseek.com/chat/completions", headers=HEADERS, data=json.dumps(data))
if response.ok:
result = response.json()
return result['choices'][0]['message']['content']
else:
raise Exception(f"Error {response.status_code}: {response.text}")
以及查询
query_deepseek(prompt)
响应如下:
"Building a web service that uses vector embeddings for search involves several components, including data processing, embedding generation, storage, search, and serving the service via an API. Below is a list of tools and technologies you can use for each step:\n\n---\n\n### 1. **Data Processing**\n - **Python**: For general data preprocessing and scripting.\n - **Pandas**: For handling tabular data.\n - **NumPy**: For numerical operations.\n - **NLTK/Spacy**: For text preprocessing (tokenization, stemming, etc.).\n - **LLM models**: For generating embeddings if you're using pre-trained models.\n\n---\n\n### 2. **Embedding Generation**\n - **Pre-trained Models**:\n - Embeddings (e.g., `text-embedding-ada-002`).\n - Hugging Face Transformers (e.g., `Sentence-BERT`, `all-MiniLM-L6-v2`).\n - Google's Universal Sentence Encoder.\n - **Custom Models**:\n - TensorFlow/PyTorch: For training custom embedding models.\n - **Libraries**:\n - `sentence-transformers`: For generating sentence embeddings.\n - `transformers`: For using Hugging Face models.\n\n---\n\n### 3. **Vector Storage**\n - **Vector Databases**:\n - Pinecone: Managed vector database for similarity search.\n - Weaviate: Open-source vector search engine.\n - Milvus: Open-source vector database.\n - FAISS (Facebook AI Similarity Search): Library for efficient similarity search.\n - Qdrant: Open-source vector search engine.\n - Redis with RedisAI: For storing and querying vectors.\n - **Traditional Databases with Vector Support**:\n - PostgreSQL with pgvector extension.\n - Elasticsearch with dense vector support.\n\n---\n\n### 4. **Search and Retrieval**\n - **Similarity Search Algorithms**:\n - Cosine similarity, Euclidean distance, or dot product for comparing vectors.\n - **Libraries**:\n - FAISS: For fast nearest-neighbor search.\n - Annoy (Approximate Nearest Neighbors Oh Yeah): For approximate nearest neighbor search.\n - **Vector Databases**: Most vector databases (e.g., Pinecone, Weaviate) come with built-in search capabilities.\n\n---\n\n### 5. **Web Service Framework**\n - **Backend Frameworks**:\n - Flask/Django/FastAPI (Python): For building RESTful APIs.\n - Node.js/Express: If you prefer JavaScript.\n - **API Documentation**:\n - Swagger/OpenAPI: For documenting your API.\n - **Authentication**:\n - OAuth2, JWT: For securing your API.\n\n---\n\n### 6. **Deployment**\n - **Containerization**:\n - Docker: For packaging your application.\n - **Orchestration**:\n - Kubernetes: For managing containers at scale.\n - **Cloud Platforms**:\n - AWS (EC2, Lambda, S3).\n - Google Cloud (Compute Engine, Cloud Functions).\n - Azure (App Service, Functions).\n - **Serverless**:\n - AWS Lambda, Google Cloud Functions, or Vercel for serverless deployment.\n\n---\n\n### 7. **Monitoring and Logging**\n - **Monitoring**:\n - Prometheus + Grafana: For monitoring performance.\n - **Logging**:\n - ELK Stack (Elasticsearch, Logstash, Kibana).\n - Fluentd.\n - **Error Tracking**:\n - Sentry.\n\n---\n\n### 8. **Frontend (Optional)**\n - **Frontend Frameworks**:\n - React, Vue.js, or Angular: For building a user interface.\n - **Libraries**:\n - Axios: For making API calls from the frontend.\n\n---\n\n### Example Workflow\n1. Preprocess your data (e.g., clean text, tokenize).\n2. Generate embeddings using a pre-trained model (e.g., Hugging Face).\n3. Store embeddings in a vector database (e.g., Pinecone or FAISS).\n4. Build a REST API using FastAPI or Flask to handle search queries.\n5. Deploy the service using Docker and Kubernetes or a serverless platform.\n6. Monitor and scale the service as needed.\n\n---\n\n### Example Tools Stack\n- **Embedding Generation**: Hugging Face `sentence-transformers`.\n- **Vector Storage**: Pinecone or FAISS.\n- **Web Framework**: FastAPI.\n- **Deployment**: Docker + AWS/GCP.\n\nBy combining these tools, you can build a scalable and efficient web service for vector embedding-based search."
8、扩展提示
虽然原始答案听起来可信,但它并没有正确回答我们的问题。相反,它只是给出了一个应用程序栈的通用描述。为了改进结果,用可用工具的描述来丰富原始提示似乎是可行的方法之一。让我们使用语义知识库,用不同技术的描述来扩充提示!
results = client.query(
collection_name="knowledge-base",
query_text=prompt,
limit=3,
)
results
响应结果如下:
[QueryResponse(id='116eb694-f127-4b80-9c94-1177ee578bba', embedding=None, sparse_embedding=None, metadata={'document': 'Qdrant is a vector database & vector similarity search engine. It deploys as an API service providing search for the nearest high-dimensional vectors. With Qdrant, embeddings or neural network encoders can be turned into full-fledged applications for matching, searching, recommending, and much more!'}, document='Qdrant is a vector database & vector similarity search engine. It deploys as an API service providing search for the nearest high-dimensional vectors. With Qdrant, embeddings or neural network encoders can be turned into full-fledged applications for matching, searching, recommending, and much more!', score=0.82907003),
QueryResponse(id='61d886cf-af3e-4ab0-bdd5-b52770832666', embedding=None, sparse_embedding=None, metadata={'document': 'FastAPI is a modern, fast (high-performance), web framework for building APIs with Python 3.7+ based on standard Python type hints.'}, document='FastAPI is a modern, fast (high-performance), web framework for building APIs with Python 3.7+ based on standard Python type hints.', score=0.81901294),
QueryResponse(id='1d904593-97a2-421b-87f4-12c9eae4c310', embedding=None, sparse_embedding=None, metadata={'document': 'PyTorch is a machine learning framework based on the Torch library, used for applications such as computer vision and natural language processing.'}, document='PyTorch is a machine learning framework based on the Torch library, used for applications such as computer vision and natural language processing.', score=0.80565226)]
我们使用原始提示对一组工具描述进行语义搜索。现在我们可以使用这些描述来扩充提示,并创建更多上下文。
context = "\n".join(r.document for r in results)
context
响应如下:
'Qdrant is a vector database & vector similarity search engine. It deploys as an API service providing search for the nearest high-dimensional vectors. With Qdrant, embeddings or neural network encoders can be turned into full-fledged applications for matching, searching, recommending, and much more!\nFastAPI is a modern, fast (high-performance), web framework for building APIs with Python 3.7+ based on standard Python type hints.\nPyTorch is a machine learning framework based on the Torch library, used for applications such as computer vision and natural language processing.'
最后,让我们构建一个元提示,它将LLM的假定角色、原始问题以及语义搜索的结果结合起来,强制LLM使用提供的上下文。
metaprompt = f"""
You are a software architect.
Answer the following question using the provided context.
If you can't find the answer, do not pretend you know it, but answer "I don't know".
Question: {prompt.strip()}
Context:
{context.strip()}
Answer:
"""
# Look at the full metaprompt
print(metaprompt)
输出如下:
You are a software architect.
Answer the following question using the provided context.
If you can't find the answer, do not pretend you know it, but answer "I don't know".
Question: What tools should I need to use to build a web service using vector embeddings for search?
Context:
Qdrant is a vector database & vector similarity search engine. It deploys as an API service providing search for the nearest high-dimensional vectors. With Qdrant, embeddings or neural network encoders can be turned into full-fledged applications for matching, searching, recommending, and much more!
FastAPI is a modern, fast (high-performance), web framework for building APIs with Python 3.7+ based on standard Python type hints.
PyTorch is a machine learning framework based on the Torch library, used for applications such as computer vision and natural language processing.
Answer:
我们目前的题目更长,并且我们还采用了一些策略来使答案更加完善:
- 大型语言模型 (LLM) 扮演软件架构师的角色。
- 我们提供更多背景信息来回答问题。
- 如果背景信息不包含任何有意义的信息,模型就不应该给出答案。
让我们看看这是否符合预期。
问题:
query_deepseek(metaprompt)
回答:
'To build a web service using vector embeddings for search, you can use the following tools:\n\n1. **Qdrant**: As a vector database and similarity search engine, Qdrant will handle the storage and retrieval of high-dimensional vectors. It provides an API service for searching and matching vectors, making it ideal for applications that require vector-based search functionality.\n\n2. **FastAPI**: This web framework is perfect for building the API layer of your web service. It is fast, easy to use, and based on Python type hints, which makes it a great choice for developing the backend of your service. FastAPI will allow you to expose endpoints that interact with Qdrant for vector search operations.\n\n3. **PyTorch**: If you need to generate vector embeddings from your data (e.g., text, images), PyTorch can be used to create and train neural network models that produce these embeddings. PyTorch is a powerful machine learning framework that supports a wide range of applications, including natural language processing and computer vision.\n\n### Summary:\n- **Qdrant** for vector storage and search.\n- **FastAPI** for building the web service API.\n- **PyTorch** for generating vector embeddings (if needed).\n\nThese tools together provide a robust stack for building a web service that leverages vector embeddings for search functionality.'
9、测试 RAG 流程
通过利用我们提供的语义上下文,我们的模型能够更好地回答问题。我们将 RAG 封装为一个函数,以便更轻松地针对不同的提示进行调用。
def rag(question: str, n_points: int = 3) -> str:
results = client.query(
collection_name="knowledge-base",
query_text=question,
limit=n_points,
)
context = "\n".join(r.document for r in results)
metaprompt = f"""
You are a software architect.
Answer the following question using the provided context.
If you can't find the answer, do not pretend you know it, but only answer "I don't know".
Question: {question.strip()}
Context:
{context.strip()}
Answer:
"""
return query_deepseek(metaprompt)
现在可以轻松地询问各种广泛的问题。
问题:
rag("What can the stack for a web api look like?")
回答:
'The stack for a web API can include the following components based on the provided context:\n\n1. **Web Framework**: FastAPI can be used as the web framework for building the API. It is modern, fast, and leverages Python type hints for better development and performance.\n\n2. **Reverse Proxy/Web Server**: NGINX can be used as a reverse proxy or web server to handle incoming HTTP requests, load balancing, and serving static content. It is known for its high performance and low resource consumption.\n\n3. **Containerization**: Docker can be used to containerize the application, making it easier to build, share, and run the API consistently across different environments without worrying about configuration issues.\n\nThis stack provides a robust, scalable, and efficient setup for building and deploying a web API.'
问题:
rag("Where is the nearest grocery store?")
回答:
"I don't know. The provided context does not contain any information about the location of the nearest grocery store."
我们的模型现在可以:
- 利用我们向量数据存储中的知识。
- 基于提供的上下文回答问题,如果无法找到答案,则明确表示“我不知道”。
原文链接:5 Minute RAG with Qdrant and DeepSeek
汇智网翻译整理,转载请标明出处
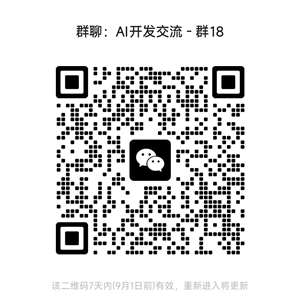