DeepSeek API+SearpApi联网搜索
DeepSeek API没有联网搜索功能,因此我们需要自己实现,这就是本教程的目的。
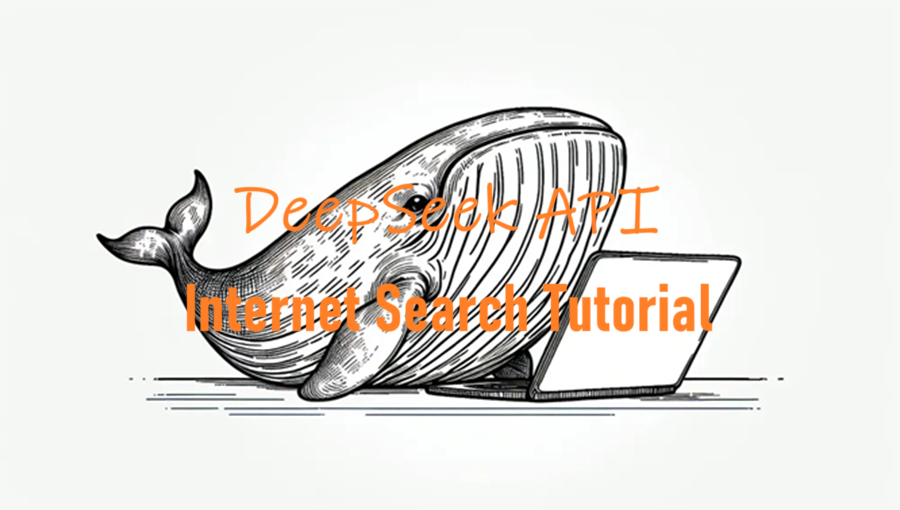
我找不到 DeepSeek 的知识截止日期,所以我直接问了 DeepSeek:
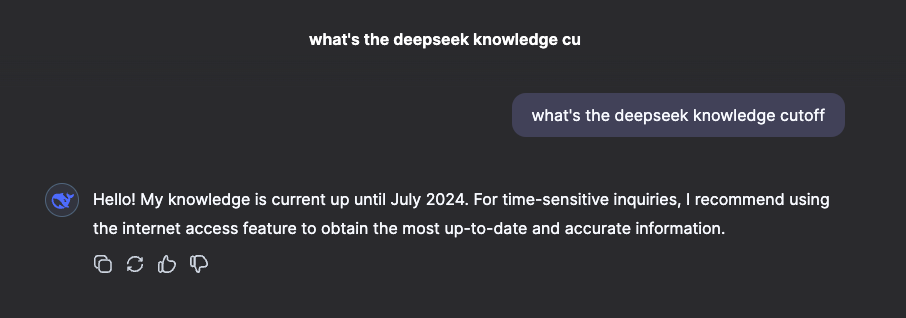
如你所见,它的最新知识来自 2024 年 7 月,它建议使用互联网访问获取最新信息。
如果你直接使用该平台,可以激活“搜索”功能来搜索网络:
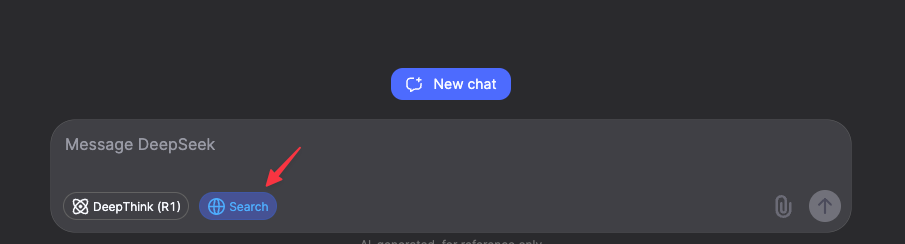
但如果使用 API,则需要找到一种变通方法,这就是我们将在本线程中讨论的内容!
1、理解 JSON 输出
我们如何理解人类语言并从中提取正确的参数调用函数?我们需要这两步:
- 从原始用户的查询中提取重要信息
- 使用提取的信息作为参数调用任何需要的函数。在上面的例子中,我们将调用另一个 API(不是 DeepSeek API),具体取决于我们要做什么。
- (可选)我们可以将我们调用的函数中的信息提供给 AI 模型,将其转换为人类语言响应。
这是第一步的模拟:
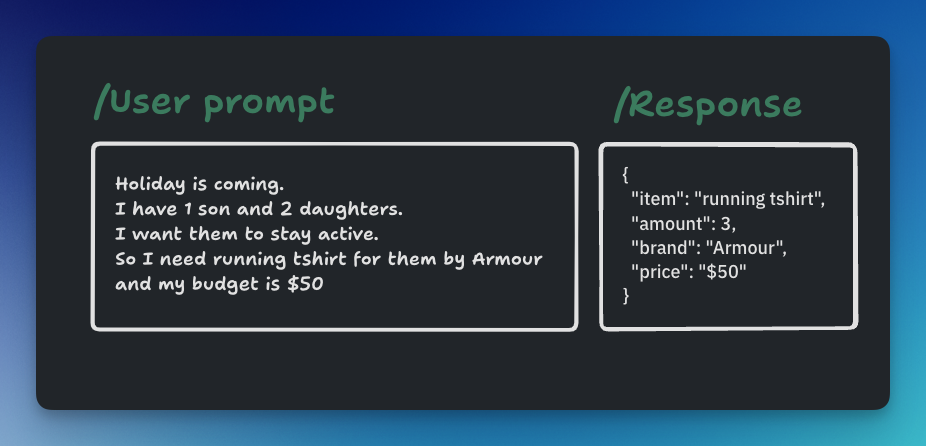
这正是 DeepSeek 的 JSON 输出功能所做的。它从人类语言返回一个很好的结构化 JSON。我们需要这种结构化的数据,以便能够用正确的参数调用自定义函数。
对于第二步,将真正取决于我们要做什么以及要调用的功能/API。对于上面的例子,我们可能想要调用一个这样的函数:
def findProducts(item, amount, brand, price):
# 一个调用 API 搜索具有这些信息的商品的方法
让我们进入示例:将 DeepSeek 与 Google 搜索结果连接!
在这个示例中,我们将向 AI 模型添加 Google 搜索功能,以便他们在回答问题之前进行 Google 搜索。这使得模型能够访问有机搜索结果、知识图谱或 Google 回答框。Google 回答框本身非常适合处理不同类型的需要实时数据的问题,比如天气:
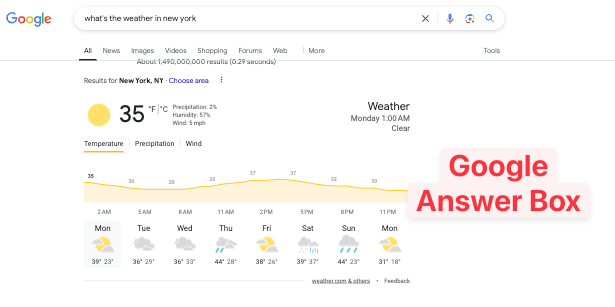
它不仅限于天气,任何 Google 可以回答的问题,DeepSeek AI 模型都能够提供!
2、DeepSeek 互联网搜索实现
你可能会认为我们需要抓取 Google 搜索结果。幸运的是,SerpApi 提供了一种简单的方法,使用 Google Search API 通过 SerpApi 轻松访问 Google 搜索信息。
你可以在游乐场免费玩一下,看看使用此 API 的结构是什么样的。
现在让我们构建程序。最终源代码可在 GitHub 上找到。
2.1 获取你的 API 密钥
在 serpapi.com 和 deepseek.com 注册以获取它们的 API 密钥。
2.2测试 SerpApi Google 搜索 API
让我们试用一下 SerpApi 的 Google 搜索 API。创建一个新的 Python 文件,文件名随意:
import requests
SERPAPI_API_KEY = "YOUR_SERPAPI_API_KEY"
def googleSearch(keyword):
url = f"https://serpapi.com/search.json?q={keyword}&api_key={SERPAPI_API_KEY}"
response = requests.get(url)
return response.json()
print(googleSearch("How to make a cake"))
现在,尝试运行此文件。确保你能看到 SerpApi 的结果。
2.3 准备 DeepSeek API
让我们准备调用 DeepSeek API 的客户端。我们将使用 OpenAI SDK。
运行以下命令安装 OpenAI SDK:
pip3 install openai
然后在之前的文件中添加以下代码:
import json
import requests
from openai import OpenAI
DEEPSEEK_API_KEY = "DEEPSEEK_API_KEY"
SERPAPI_API_KEY = "SERPAPI_API_KEY"
def googleSearch(keyword):
url = f"https://serpapi.com/search.json?q={keyword}&api_key={SERPAPI_API_KEY}"
response = requests.get(url)
return response.json()
# 添加 DeepSeek 客户端
client = OpenAI(
api_key=DEEPSEEK_API_KEY,
base_url="https://api.deepseek.com",
)
2.4 JSON 输出功能
现在,我们将测试 DeepSeek 的 JSON 输出功能,该功能能够从人类语言中提取重要信息。在此步骤中,我们不会调用 googleSearch
方法。
client = OpenAI(
api_key=DEEPSEEK_API_KEY,
base_url="https://api.deepseek.com",
)
# Basic sample for DeepSeek JSON Output
system_prompt = """
Please parse the "keyword" from user's message to be used in a Google search and output them in JSON format.
EXAMPLE INPUT:
What's the weather like in New York today?
EXAMPLE JSON OUTPUT:
{
"keyword": "weather in New York"
}
"""
user_prompt = "What's the weather like in London today?"
messages = [{"role": "system", "content": system_prompt},
{"role": "user", "content": user_prompt}]
response = client.chat.completions.create(
model="deepseek-chat",
messages=messages,
response_format={
'type': 'json_object'
}
)
print(json.loads(response.choices[0].message.content))
- 提供一个系统提示,告诉 AI 模型应该做什么。该提示必须包含 "JSON" 关键字。
- 添加用户提示。它可以是任意内容。
- 使用上述响应格式调用聊天完成方法。
以下是输出:
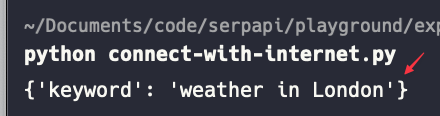
让我们尝试其他方法。我们将用以下内容替换用户提示:
user_prompt = "Can you please check Tesla's stock price today?"
它返回:
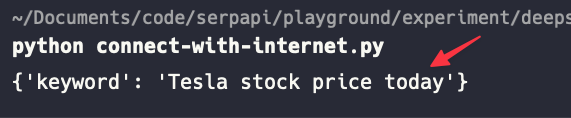
正如你所见,AI 模型足够聪明,可以从用户提示中提取信息,并将其转换为一个我们可以搜索的关键字。
2.5 将 DeepSeek 连接到外部函数
现在进入有趣的部分,让我们将之前准备好的谷歌搜索方法与我们提取的关键字连接起来。
response = client.chat.completions.create(
model="deepseek-chat",
messages=messages,
response_format={
'type': 'json_object'
}
)
keyword = json.loads(response.choices[0].message.content)["keyword"]
googleResponse = googleSearch(keyword)
print(json.dumps(googleResponse, indent=4)) #I wrap it on json.dumps to make it easier to see
谷歌搜索 API 返回了大量信息,但是我们只对 answer_box 部分感兴趣。正如你所见,它包含了当前的股票价格信息,这正是我们需要的。
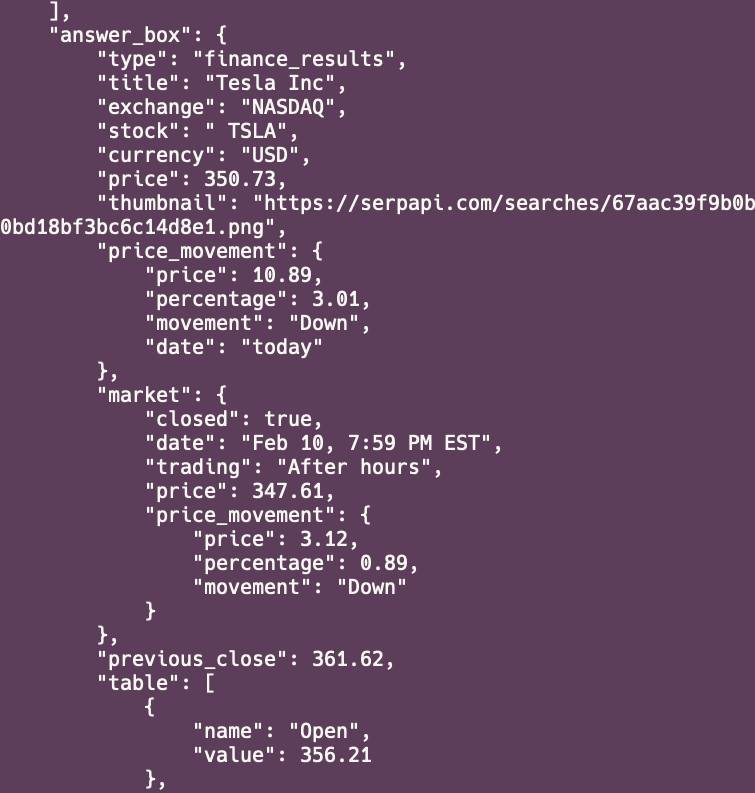
2.6 提供自然语言响应
我们可以通过仅返回价格信息来停止程序,但我们可以通过用人类自然语言返回信息来提高用户体验。
为了实现这一点,我们需要将这些信息发送给 AI 并让它回答问题。
警告:为了避免使用过多的令牌,我们应该只将所需的信息传递给 AI 模型,而不是整个响应。
这是代码的样子:
response = client.chat.completions.create(
model="deepseek-chat",
messages=messages,
response_format={
'type': 'json_object'
}
)
keyword = json.loads(response.choices[0].message.content)["keyword"]
googleResponse = googleSearch(keyword)
answer_box = googleResponse["answer_box"]
answer_box_to_string = json.dumps(answer_box, indent=2)
if answer_box:
response = client.chat.completions.create(
model="deepseek-chat",
messages=[
{"role": "system", "content": "Answer the question from user with provided information: " + answer_box_to_string},
{"role": "user", "content": user_prompt},
]
)
print(response.choices[0].message.content)
else:
print("No answer box found")
我们调出另一个聊天补全方法来用人类语言回答问题。以下是响应:

我们可以通过将其与实际的谷歌搜索结果进行比较来验证这些信息是真实的:
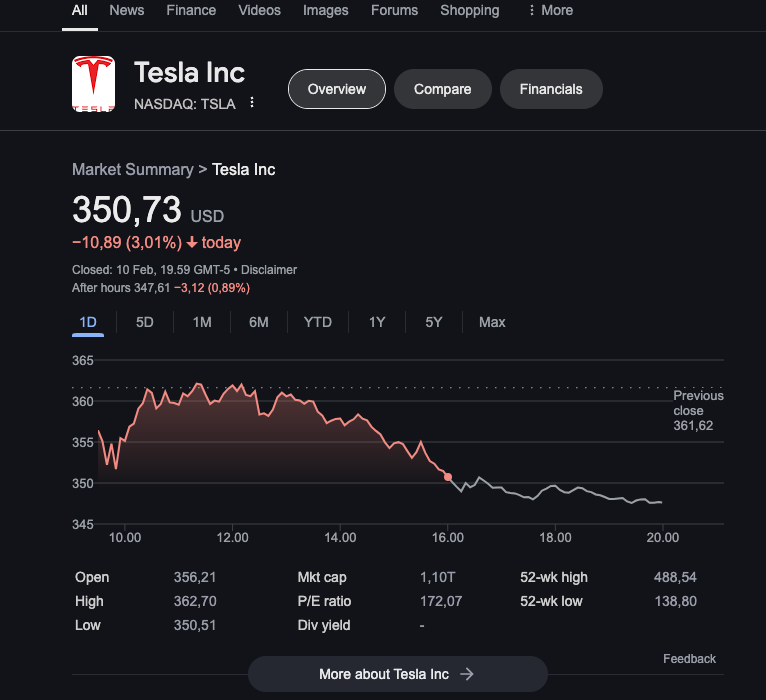
想象一下,现在我们可以将实时数据添加到我们的 DeepSeek AI 模型中!
现在我们可以做的事情多了!
3、DeepSeek 地图推荐实现
让我们来看看另一个案例。假设我们想根据用户的说法在 Google 地图上找到地点。
步骤与前面的示例相似,但这次,我们将使用 Google 地图 API 进行额外的功能调用。
最终源代码可在 GitHub 上找到。
3.1 Google 地图 API 方法
这是通过 SerpApi 访问 Google 地图 API 的方法:
def mapsSearch(keyword):
url = f"https://serpapi.com/search.json?engine=google_maps&q={keyword}&api_key={SERPAPI_API_KEY}"
response = requests.get(url)
return response.json()
3.2 调整系统提示
根据需要调整系统提示:
system_prompt = """
Please parse the "keyword" from user's message to be used in a Google Maps and output them in JSON format.
EXAMPLE INPUT:
I'm hungry, not sure what's to eat?
EXAMPLE JSON OUTPUT:
{
"keyword": "restaurant"
}
OTHER EXAMPLE INPUT:
I'm craving some sushi right now
OTHER EXAMPLE JSON OUTPUT:
{
"keyword": "sushi restaurant"
}
"""
3.3 测试 DeepSeek JSON 输出
确保 JSON 输出正确工作,尝试运行此脚本:
user_prompt = "Really craving some Korean BBQ right now"
messages = [
{"role": "system", "content": system_prompt},
{"role": "user", "content": user_prompt}
]
response = client.chat.completions.create(
model="deepseek-chat",
messages=messages,
response_format={
'type': 'json_object'
}
)
keyword = json.loads(response.choices[0].message.content)["keyword"]
print(keyword)
我得到了"Korean BBQ restaurant"。
3.4 连接 Google 地图 API
现在我们能够从用户那里检索到正确的关键字,让我们调用之前准备的 mapsSearch
函数:
user_prompt = "Really craving some Korean BBQ right now"
messages = [
{"role": "system", "content": system_prompt},
{"role": "user", "content": user_prompt}
]
response = client.chat.completions.create(
model="deepseek-chat",
messages=messages,
response_format={
'type': 'json_object'
}
)
keyword = json.loads(response.choices[0].message.content)["keyword"]
mapsResult = mapsSearch(keyword)
if mapsResult["local_results"]:
top_places = mapsResult["local_results"][:3]
response = client.chat.completions.create(
model="deepseek-chat",
messages=[
{"role": "system", "content": "Answer the user with top 3 places informations: " + json.dumps(top_places, indent=2)},
{"role": "user", "content": user_prompt},
]
)
print(response.choices[0].message.content)
else:
print("No local results found")
解释:
- 我们将地图结果存储在
mapsResult
变量中 - 我们只抓取前 3 个结果,根据你的用例可以自由调整
- 调用 AI 模型以人类语言响应并提供前三个地点信息
结果如下:
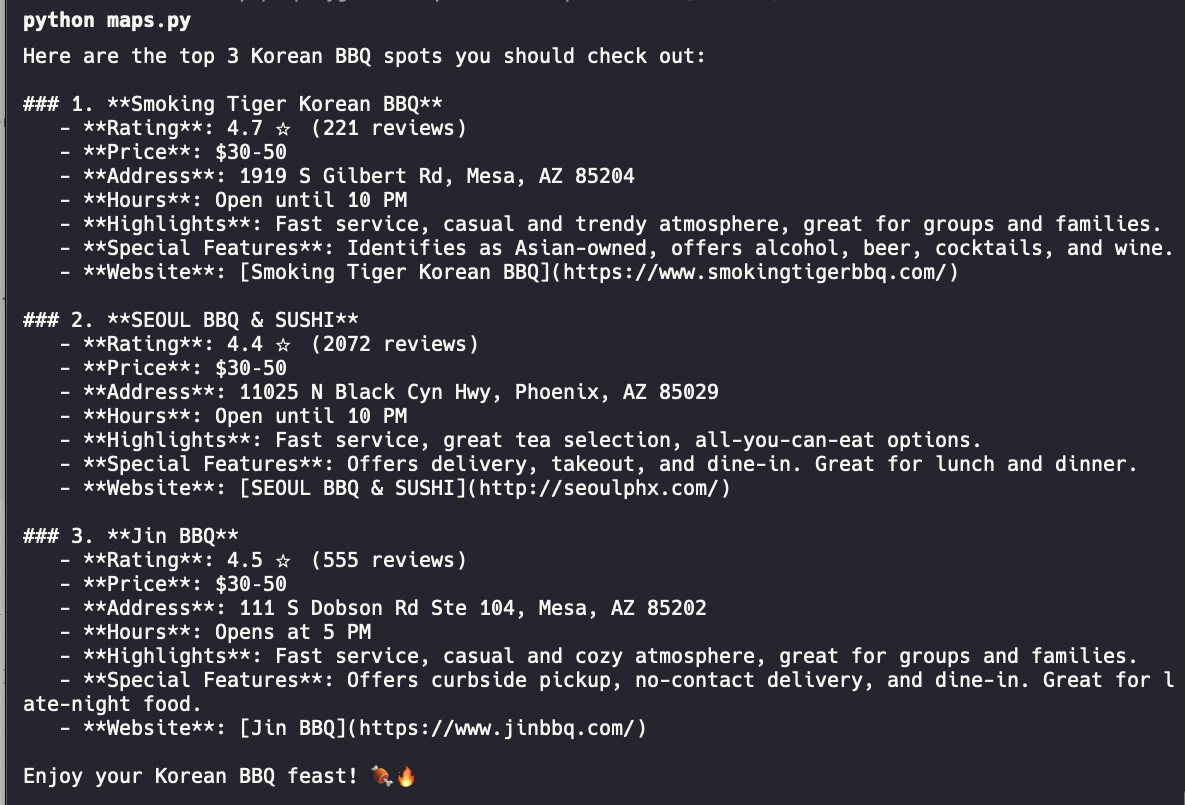
4、函数调用 vs JSON 输出
我们有两种主要方法使用 DeepSeek API 调用另一个函数:函数调用和 JSON 输出。虽然这些方法不便于直接进行互联网调用,但它们有助于从原始字符串中提取重要信息,然后用于调用外部函数。
函数调用专注于执行由 API 提供的特定功能,而 JSON 输出强调以可读格式进行结构化数据交换。需要注意的是,目前函数调用方法不稳定。这就是为什么在本教程中我们将使用 JSON 输出方法。
参考资料:
- 函数调用:https://api-docs.deepseek.com/guides/function_calling
- JSON 输出:https://api-docs.deepseek.com/guides/json_mode
如果你对基本的 DeepSeek API 感兴趣,请参阅这篇帖子:探索 DeepSeek API 。
新的人工智能竞争对手已经到来。探索 DeepSeek API:聊天完成、JSON 输出、函数调用、多轮对话等更多功能!
原文链接:Connect DeepSeek API with real-time data from the Internet
汇智网翻译整理,转载请标明出处
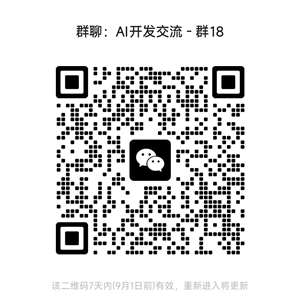