Docling 文档提取简明教程
Docling是IBM 深度搜索团队的 MIT 许可文档提取 Python 库,可轻松快速地解析文档并将其导出为所需格式。
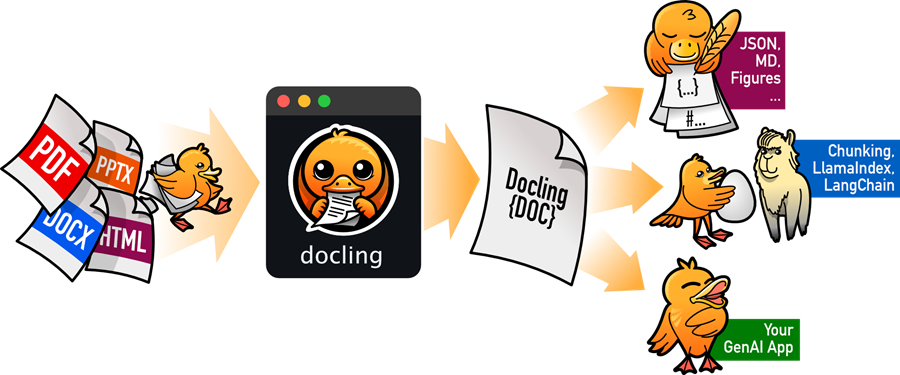
Docling是IBM 深度搜索团队的 MIT 许可文档提取 Python 库,可轻松快速地解析文档并将其导出为所需格式。
Docling的功能包括:
- 读取流行的文档格式(PDF、DOCX、PPTX、图像、HTML、AsciiDoc、Markdown)并导出为 Markdown 和 JSON
- 高级 PDF 文档理解,包括页面布局、阅读顺序和表格结构
- 统一、富有表现力的 DoclingDocument 表示格式
- 元数据提取,包括标题、作者、参考文献和语言
- 无缝 LlamaIndex 🦙 和 LangChain 🦜🔗 集成,打造强大的 RAG / QA 应用程序
- 扫描 PDF 的 OCR 支持
- 简单方便的 CLI
1、Docling安装
要使用 Docling,只需从 Python 包管理器安装 docling,例如 pip:
pip install docling
以上命令适用于 macOS、Linux 和 Windows,支持 x86_64 和 arm64 架构。
如果你希望开发 Docling 功能、错误修复等,请从本地克隆的根目录按如下方式安装:
poetry install --all-extras
2、用docling转换单个文档
要转换单个 PDF 文档,请使用 convert()
,例如:
from docling.document_converter import DocumentConverter
source = "https://arxiv.org/pdf/2408.09869" # PDF path or URL
converter = DocumentConverter()
result = converter.convert(source)
print(result.document.export_to_markdown()) # output: "### Docling Technical Report[...]"
你还可以直接从命令行使用 Docling 转换单个文件(无论是本地文件还是通过 URL)或整个目录。
一个简单的示例如下所示:
docling https://arxiv.org/pdf/2206.01062
要查看所有可用选项(导出格式等),请运行 docling --help
。
3、高级选项
示例文件 custom_convert.py 包含多种调整转换管道和功能的方法。
3.1 控制 PDF 表格提取选项
你可以控制表格结构识别是否应将识别的结构映射回 PDF 单元格(默认)或使用结构预测本身的文本单元格。如果你发现提取的表格中的多列被错误地合并为一列,这可以提高输出质量。
from docling.datamodel.base_models import InputFormat
from docling.document_converter import DocumentConverter, PdfFormatOption
from docling.datamodel.pipeline_options import PdfPipelineOptions
pipeline_options = PdfPipelineOptions(do_table_structure=True)
pipeline_options.table_structure_options.do_cell_matching = False # uses text cells predicted from table structure model
doc_converter = DocumentConverter(
format_options={
InputFormat.PDF: PdfFormatOption(pipeline_options=pipeline_options)
}
)
自 docling 1.16.0 起:您可以控制要使用的 TableFormer 模式。在 TableFormerMode.FAST(默认)和 TableFormerMode.ACCURATE(更好,但更慢)之间进行选择,以获得更高质量的复杂表格结构。
from docling.datamodel.base_models import InputFormat
from docling.document_converter import DocumentConverter, PdfFormatOption
from docling.datamodel.pipeline_options import PdfPipelineOptions, TableFormerMode
pipeline_options = PdfPipelineOptions(do_table_structure=True)
pipeline_options.table_structure_options.mode = TableFormerMode.ACCURATE # use more accurate TableFormer model
doc_converter = DocumentConverter(
format_options={
InputFormat.PDF: PdfFormatOption(pipeline_options=pipeline_options)
}
)
3.2 提供特定的工件路径
默认情况下,模型等工件在首次使用时会自动下载。如果您你望使用已明确预取工件的本地路径,则可以按如下方式执行:
from docling.datamodel.base_models import InputFormat
from docling.datamodel.pipeline_options import PdfPipelineOptions
from docling.document_converter import DocumentConverter, PdfFormatOption
from docling.pipeline.standard_pdf_pipeline import StandardPdfPipeline
# # to explicitly prefetch:
# artifacts_path = StandardPdfPipeline.download_models_hf()
artifacts_path = "/local/path/to/artifacts"
pipeline_options = PdfPipelineOptions(artifacts_path=artifacts_path)
doc_converter = DocumentConverter(
format_options={
InputFormat.PDF: PdfFormatOption(pipeline_options=pipeline_options)
}
)
3.3 对文档大小施加限制
你可以限制每个文档应允许处理的文件大小和页数:
from pathlib import Path
from docling.document_converter import DocumentConverter
source = "https://arxiv.org/pdf/2408.09869"
converter = DocumentConverter()
result = converter.convert(source, max_num_pages=100, max_file_size=20971520)
3.4 从二进制 PDF 流转换
你可以从二进制流而不是文件系统转换 PDF,如下所示:
from io import BytesIO
from docling.datamodel.base_models import DocumentStream
from docling.document_converter import DocumentConverter
buf = BytesIO(your_binary_stream)
source = DocumentStream(filename="my_doc.pdf", stream=buf)
converter = DocumentConverter()
result = converter.convert(source)
3.5 限制资源使用量
你可以通过相应地设置环境变量 OMP_NUM_THREADS
来限制 Docling 使用的 CPU 线程。默认设置是使用 4 个 CPU 线程。
4、Docling文档分块
你可以按如下方式对 Docling 文档执行层次结构感知分块:
from docling.document_converter import DocumentConverter
from docling_core.transforms.chunker import HierarchicalChunker
conv_res = DocumentConverter().convert("https://arxiv.org/pdf/2206.01062")
doc = conv_res.document
chunks = list(HierarchicalChunker().chunk(doc))
print(chunks[30])
# {
# "text": "Lately, new types of ML models for document-layout analysis have emerged [...]",
# "meta": {
# "doc_items": [{
# "self_ref": "#/texts/40",
# "label": "text",
# "prov": [{
# "page_no": 2,
# "bbox": {"l": 317.06, "t": 325.81, "r": 559.18, "b": 239.97, ...},
# }]
# }],
# "headings": ["2 RELATED WORK"],
# }
# }
5、Docling v2
Docling v2 引入了几个新功能:
- 理解并转换 PDF、MS Word、MS Powerpoint、HTML 和多种图像格式
- 生成一种新的通用文档表示,可以封装文档层次结构
- 附带全新的 API 和 CLI
5.1 CLI变化
我们更新了 Docling v2 的命令行语法以支持多种格式。示例如下。
# Convert a single file to Markdown (default)
docling myfile.pdf
# Convert a single file to Markdown and JSON, without OCR
docling myfile.pdf --to json --to md --no-ocr
# Convert PDF files in input directory to Markdown (default)
docling ./input/dir --from pdf
# Convert PDF and Word files in input directory to Markdown and JSON
docling ./input/dir --from pdf --from docx --to md --to json --output ./scratch
# Convert all supported files in input directory to Markdown, but abort on first error
docling ./input/dir --output ./scratch --abort-on-error
与 Docling v1 相比的显著变化:
- 删除了用于不同导出格式的独立开关,并用 --from 和 --to 参数替换,分别定义输入和输出格式。
- 新的 --abort-on-error 将在遇到错误时立即中止任何批量转换
- PDF 的 --backend 选项已被删除
5.2 设置 DocumentConverter
为了适应多种输入格式,我们改变了设置 DocumentConverter 对象的方式。您现在可以在 DocumentConverter 初始化时定义允许的格式列表,并根据需要为每种格式指定自定义选项。默认情况下,允许所有支持的格式。如果您不提供 format_options,则将对所有 allowed_formats 使用默认值。
格式选项可以包括要使用的管道类、要提供给管道的选项以及文档后端。它们以格式特定的类型提供,例如 PdfFormatOption 或 WordFormatOption,如下所示。
from docling.document_converter import DocumentConverter
from docling.datamodel.base_models import InputFormat
from docling.document_converter import (
DocumentConverter,
PdfFormatOption,
WordFormatOption,
)
from docling.pipeline.simple_pipeline import SimplePipeline
from docling.pipeline.standard_pdf_pipeline import StandardPdfPipeline
from docling.datamodel.pipeline_options import PdfPipelineOptions
from docling.backend.pypdfium2_backend import PyPdfiumDocumentBackend
## Default initialization still works as before:
# doc_converter = DocumentConverter()
# previous `PipelineOptions` is now `PdfPipelineOptions`
pipeline_options = PdfPipelineOptions()
pipeline_options.do_ocr = False
pipeline_options.do_table_structure = True
#...
## Custom options are now defined per format.
doc_converter = (
DocumentConverter( # all of the below is optional, has internal defaults.
allowed_formats=[
InputFormat.PDF,
InputFormat.IMAGE,
InputFormat.DOCX,
InputFormat.HTML,
InputFormat.PPTX,
], # whitelist formats, non-matching files are ignored.
format_options={
InputFormat.PDF: PdfFormatOption(
pipeline_options=pipeline_options, # pipeline options go here.
backend=PyPdfiumDocumentBackend # optional: pick an alternative backend
),
InputFormat.DOCX: WordFormatOption(
pipeline_cls=SimplePipeline # default for office formats and HTML
),
},
)
)
注意:如果你仅使用默认值,则所有内容与 Docling v1 中的内容相同。
以下示例单元显示了更多选项:
- run_with_formats.py
- custom_convert.py
5.3 转换文档
我们简化了向 DocumentConverter 输入输入的方式,并重命名了转换方法以获得更好的语义。现在,您可以直接使用单个文件、输入文件列表或 DocumentStream 对象调用转换,而无需先构造 DocumentConversionInput 对象。
- DocumentConverter.convert 现在转换单个文件输入(以前为 DocumentConverter.convert_single)。
- DocumentConverter.convert_all 现在可以一次转换多个文件(以前为 DocumentConverter.convert)。
...
from docling.datamodel.document import ConversionResult
## Convert a single file (from URL or local path)
conv_result: ConversionResult = doc_converter.convert("https://arxiv.org/pdf/2408.09869") # previously `convert_single`
## Convert several files at once:
input_files = [
"tests/data/wiki_duck.html",
"tests/data/word_sample.docx",
"tests/data/lorem_ipsum.docx",
"tests/data/powerpoint_sample.pptx",
"tests/data/2305.03393v1-pg9-img.png",
"tests/data/2206.01062.pdf",
]
# Directly pass list of files or streams to `convert_all`
conv_results_iter = doc_converter.convert_all(input_files) # previously `convert`
通过 raises_on_error 参数,您还可以控制转换是否在第一次遇到问题时引发异常,或者首先弹性地转换所有文件并在每个文件中反映错误
...
conv_results_iter = doc_converter.convert_all(input_files, raises_on_error=False) # previously `convert`
5.4 访问文档结构
我们还简化了访问和导出转换后的文档数据的方式。我们的通用文档表示现在可作为 DoclingDocument 对象在转换结果中使用。DoclingDocument 提供了一组简洁的 API 来构建、迭代和导出文档中的内容,如下所示。
conv_result: ConversionResult = doc_converter.convert("https://arxiv.org/pdf/2408.09869") # previously `convert_single`
## Inspect the converted document:
conv_result.document.print_element_tree()
## Iterate the elements in reading order, including hierachy level:
for item, level in conv_result.document.iterate_items:
if isinstance(item, TextItem):
print(item.text)
elif isinstance(item, TableItem):
table_df: pd.DataFrame = item.export_to_dataframe()
print(table_df.to_markdown())
elif ...:
#...
注意:虽然它已被弃用,但您仍然可以使用 Docling v1 文档表示,它可用作:
conv_result.legacy_document # provides the representation in previous ExportedCCSDocument type
5.5 导出为 JSON、Markdown、Doctags
注意:ConversionResult 中的所有 render_... 方法已在 Docling v2 中删除,现在可在 DoclingDocument 上使用:
- DoclingDocument.export_to_dict
- DoclingDocument.export_to_markdown
- DoclingDocument.export_to_document_tokens
conv_result: ConversionResult = doc_converter.convert("https://arxiv.org/pdf/2408.09869") # previously `convert_single`
## Export to desired format:
print(json.dumps(conv_res.document.export_to_dict()))
print(conv_res.document.export_to_markdown())
print(conv_res.document.export_to_document_tokens())
注意:虽然它已被弃用,但您仍然可以导出 Docling v1 JSON 格式。这可通过与 DoclingDocument 类型相同的方法获得:
## Export legacy document representation to desired format, for v1 compatibility:
print(json.dumps(conv_res.legacy_document.export_to_dict()))
print(conv_res.legacy_document.export_to_markdown())
print(conv_res.legacy_document.export_to_document_tokens())
5.6 重新加载存储为 JSON 的 DoclingDocument
您可以使用以下代码以 JSON 格式将 DoclingDocument 保存并重新加载到磁盘:
# Save to disk:
doc: DoclingDocument = conv_res.document # produced from conversion result...
with Path("./doc.json").open("w") as fp:
fp.write(json.dumps(doc.export_to_dict())) # use `export_to_dict` to ensure consistency
# Load from disk:
with Path("./doc.json").open("r") as fp:
doc_dict = json.loads(fp.read())
doc = DoclingDocument.model_validate(doc_dict) # use standard pydantic API to populate doc
5.7 分块
Docling v2 为分块定义了新的基类:
- BaseMeta 用于分块元数据
- BaseChunk 包含分块文本和元数据,以及
- BaseChunker 用于分块器,从 DoclingDocument 中生成分块。
此外,它还提供了更新的 HierarchicalChunker 实现,它利用新的 DoclingDocument 并提供一种新的、更丰富的分块输出格式,包括:
- 用于基础的各个文档项
- 任何适用于上下文的标题
- 任何适用于上下文的说明
有关示例,请查看分块用法。
原文链接:Docling - get your documents ready for gen AI
汇智网翻译整理,转载请标明出处