哼唱搜歌原理及实现
想象一下,你哼唱的歌曲直接出现在屏幕上?这就是我们今天要做的。
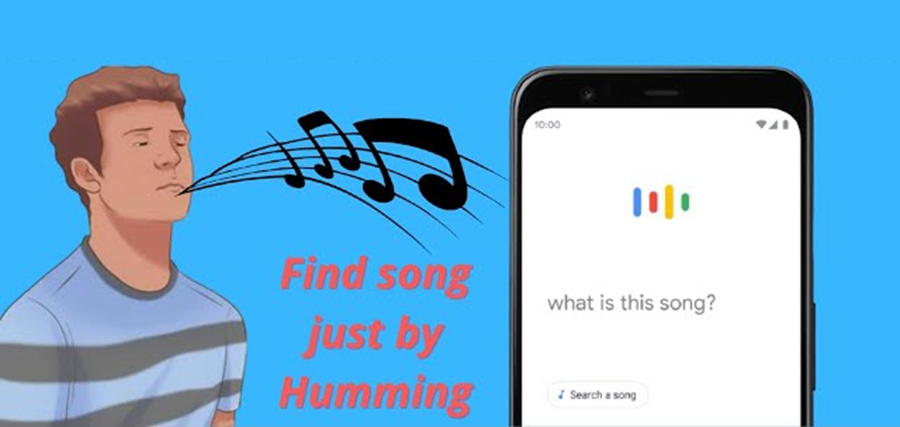
欢迎来到音乐信息检索的未来,机器学习、矢量数据库和音频数据分析融合在一起,提供令人兴奋的新可能性!如果你对音乐数据分析的世界感兴趣,或者只是对技术如何彻底改变音乐行业充满热情,那么本指南适合你。
在这里,我们将带你踏上使用称为向量搜索的方法搜索音乐数据的旅程。由于我们世界上 80% 以上的数据都是非结构化的,因此了解如何处理除文本以外的不同类型的数据是很好的。
如果你想在阅读时关注并执行代码,请访问 GitHub 仓库。
1、系统架构
想象一下,如果你可以哼唱你想回忆的歌曲的曲调,突然间你哼唱的歌曲出现在屏幕上?当然,只要付出必要的努力和数据模型调整,这就是我们今天要做的。
为了实现我们的结果,我们将创建一个如下所示的架构:
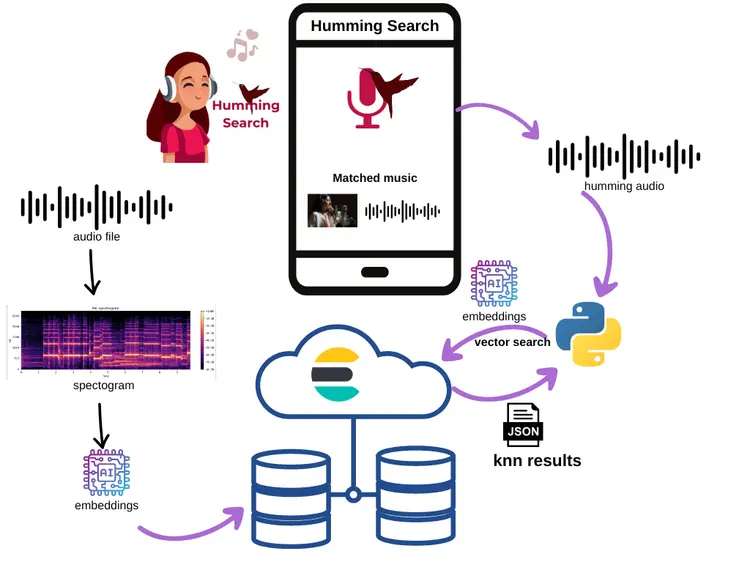
这里的主要角色是嵌入(embedding)。我们将使用模型生成的音频嵌入作为向量搜索中的搜索关键字。
2、如何生成音频嵌入
生成嵌入的核心是经过数百万个示例训练的模型,以提供更相关、更准确的结果。 对于音频,这些模型可以在大量音频数据上进行训练。 这些模型的输出是音频的密集数字表示(即音频嵌入)。 这个高维向量捕获音频片段的关键特征,允许在嵌入空间中进行相似度计算和高效搜索。
对于这项工作,我们将使用 librosa(开源 Python 包)来生成音频嵌入。 这通常涉及从音频文件中提取有意义的特征,例如梅尔频率倒谱系数 (MFCC)、色度(chroma)和梅尔缩放频谱图特征。 那么,我们如何使用 Elasticsearch 实现音频搜索?
3、创建索引以存储音频数据
首先,我们需要在 Elasticsearch 中创建索引,然后再用音乐数据填充矢量数据库。
- 首先部署 Elasticsearch。
- 在此过程中,请记住存储要在我们的 Python 代码中使用的凭据(用户名、密码)。
- 为简单起见,我们将使用在 Jupyter Notebook(Google Collab)上运行的 Python 代码。
现在我们有了连接,让我们创建一个用于存储音频信息的索引。
# Here we're defining the index configuration. We're setting up mappings for our index which determine the data types for the fields in our documents.
index_config = {
"mappings": {
"_source": {
"excludes": ["audio-embedding"]
},
"properties": {
"audio-embedding": {
"type": "dense_vector",
"dims": 2048,
"index": True,
"similarity": "cosine"
},
"path": {
"type": "text",
"fields": {
"keyword": {
"type": "keyword",
"ignore_above": 256
}
}
},
"timestamp": {
"type": "date"
},
"title": {
"type": "text"
}
}
}
}
# Index name for creating in Elasticsearch
index_name = "my-audio-index"
# Checking and creating the index
if not es.indices.exists(index=index_name):
index_creation = es.indices.create(index=index_name, ignore=400, body=index_config)
print("index created: ", index_creation)
else:
print("Index already exists.")
提供的 Python 代码使用 Elasticsearch Python 客户端创建具有特定配置的索引。索引的目的是提供一种结构,允许在密集向量字段上进行搜索操作,这些字段通常用于存储某些实体(例如本例中的音频文件)的向量表示或嵌入。
index_config
对象定义此索引的映射属性,包括“audio-embedding”、“path”、“timestamp”和“title”字段。“audio-embedding”字段被指定为“dense_vector”类型,可容纳 2048 维,并使用“余弦”相似性进行索引,这决定了在搜索操作期间用于计算向量之间距离的方法。“path”字段将存储播放音频的路径。请注意,要适应 2048 的嵌入维数,您需要使用 Elasticsearch 版本 8.8.0 或更高版本。
然后,脚本检查 Elasticsearch 实例中是否存在索引。如果索引不存在,它会创建一个具有指定配置的新索引。这种类型的索引配置可用于音频搜索等场景,其中音频文件被转换为矢量表示以进行索引和随后的基于相似性的检索。
4、用音频数据填充 Elasticsearch
在此步骤结束时,你将读取一个索引,用音频数据填充以创建我们的数据存储。为了继续进行音频搜索,我们首先需要填充我们的数据库。
4.1 选择要提取的音频数据
许多音频数据集都有特定的目标。对于我们的示例,我将使用在 Google Music LM 页面上生成的文件,特别是来自文本和旋律调节部分的文件。将你的音频文件 *.wav 放在特定目录中 — 在本例中,我选择我的 GoogleDrive“/content/drive/MyDrive/audios”。
import os
def list_audio_files(directory):
# The list to store the names of .mp3, .wav files
audio_files = []
# Check if the path exists
if os.path.exists(directory):
# Walk the directory
for root, dirs, files in os.walk(directory):
for file in files:
# Check if the file is a .wav file
if file.endswith('.wav'):
# Extract the filename from the path
filename = os.path.splitext(file)[0]
print(filename)
# Add the file to the list
audio_files.append(file)
else:
print(f"The directory '{directory}' does not exist.")
# Return the list of .mp3 files
return audio_files
# Use the function
audio_files = list_audio_files("/content/drive/MyDrive/audios")
代码定义了一个名为 list_audio_files
的函数,该函数以目录为参数。此函数旨在遍历提供的目录及其子目录,查找扩展名为“.wav”的音频文件。如果需要支持 .mp3 文件,则需要修改该函数。
4.2 向量搜索的嵌入功能
这一步是奇迹发生的地方。向量相似性搜索是一种根据给定查询的相似性来存储、检索和搜索向量的机制,常用于图像检索、自然语言处理、推荐系统等应用。由于深度学习的兴起和使用嵌入表示数据,这一概念得到了广泛应用。本质上,嵌入是高维数据的向量表示。
基本思想是将数据项(例如图像、文档、用户个人资料)表示为高维空间中的向量。然后,使用距离度量(例如余弦相似度或欧几里得距离)测量向量之间的相似度,并返回最相似的向量作为搜索结果。虽然文本嵌入是使用语言特征提取的,但音频嵌入通常使用频谱图或其他音频信号特征生成。
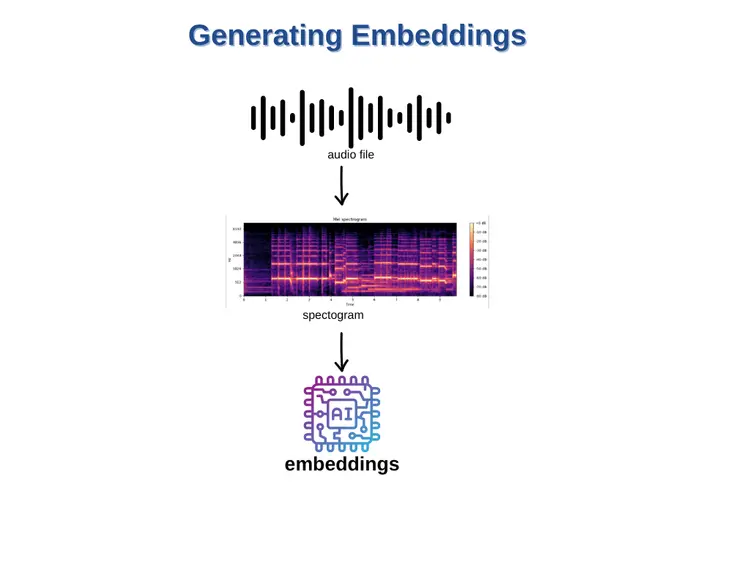
4.3 提取音频特征
下一步涉及分析我们的音频文件并提取有意义的特征。此步骤至关重要,因为它有助于机器学习模型理解和学习我们的音频数据。
在机器学习的音频信号处理中,从频谱图中提取特征的过程是至关重要的一步。频谱图是音频信号随时间变化的频率内容的视觉表示。在此背景下识别的特征包括三种特定类型:
- 梅尔频率倒谱系数 (MFCC):MFCC 是以与人类听觉感知更密切相关的方式捕获音频信号频谱特征的系数。
- 色度(chroma)特征:色度特征代表音乐八度的 12 个不同音高类别,在音乐相关任务中特别有用。
- 频谱对比:频谱对比关注音频信号中不同频带的感知亮度。
通过分析和比较这些特征集在真实文本文件中的有效性,研究人员和从业者可以获得有价值的见解,了解它们是否适用于各种基于音频的机器学习应用,例如音频分类和分析。
- 首先,我们需要将音频文件转换为适合分析的格式。Python 中的 librosa 等库可以帮助进行这种转换,将音频文件转换为频谱图。
- 接下来,我们将从这些频谱图中提取特征。
- 然后,我们将保存这些特征并将它们作为输入发送到我们的机器学习模型。
我们正在使用 panns_inference,这是一个专为音频标记和声音事件检测任务而设计的 Python 库。该库中使用的模型是从 PANN 训练的,PANN 代表大规模预训练音频神经网络,是一种音频模式识别方法。
!pip install -qU panns-inference librosa
from panns_inference import AudioTagging
# load the default model into the gpu.
model = AudioTagging(checkpoint_path=None, device='cuda') # change device to cpu if a gpu is not available
注意:下载 PANNS 推理模型可能需要几分钟。
import numpy as np
# Function to normalize a vector. Normalizing a vector
means adjusting the values measured in different scales
to a common scale.
def normalize(v):
# np.linalg.norm computes the vector's norm (magnitude).
The norm is the total length of all vectors in a space.
norm = np.linalg.norm(v)
if norm == 0:
return v
# Return the normalized vector.
return v / norm
# Function to get an embedding of an audio file. An embedding is a reduced-dimensionality representation of the file.
def get_embedding (audio_file):
# Load the audio file using librosa's load function, which returns an audio time series and its corresponding sample rate.
a, _ = librosa.load(audio_file, sr=44100)
# Reshape the audio time series to have an extra dimension, which is required by the model's inference function.
query_audio = a[None, :]
# Perform inference on the reshaped audio using the model. This returns an embedding of the audio.
_, emb = model.inference(query_audio)
# Normalize the embedding. This scales the embedding to have a length (magnitude) of 1, while maintaining its direction.
normalized_v = normalize(emb[0])
# Return the normalized embedding required for dot_product elastic similarity dense vector
return normalized_v
4.4 将音频数据插入 Elasticsearch
现在我们已经拥有将音频数据插入 Elasticsearch 索引所需的一切。
from datetime import datetime
#Storing Songs in Elasticsearch with Vector Embeddings:
def store_in_elasticsearch(song, embedding, path, index_name, genre, vec_field):
body = {
'audio-embedding' : embedding,
'title': song,
'timestamp': datetime.now(),
'path' : path,
'genre' : genre
}
es.index(index=index_name, document=body)
print ("stored...",song, embedding, path, genre, index_name)
audio_path = "/content/drive/MyDrive/@Blogs/MusicSearch/audios/"
# Initialize a list genre for test
genre_lst = ['jazz', 'opera', 'piano','prompt', 'humming', 'string', 'capella', 'eletronic', 'guitar']
for filename in audio_files:
audio_file = audio_path + filename
emb = get_embedding(audio_file)
song = filename
# Compare if genre list exists inside the song
for g in genre_lst:
if g in song.lower():
genre = g
else:
genre = "generic"
store_in_elasticsearch(song, emb, audio_file, index_name, genre, 2 )
4.5 在 Kibana 中可视化结果
此时,我们可以使用嵌入音频嵌入密集矢量场的音频数据检查索引。Kibana Dev Tools(尤其是控制台功能)是一个强大的界面,可用于与 Elasticsearch 集群进行交互。它提供了一种直接向 Elasticsearch 发送 RESTful 命令并以用户友好的格式查看结果的方法。
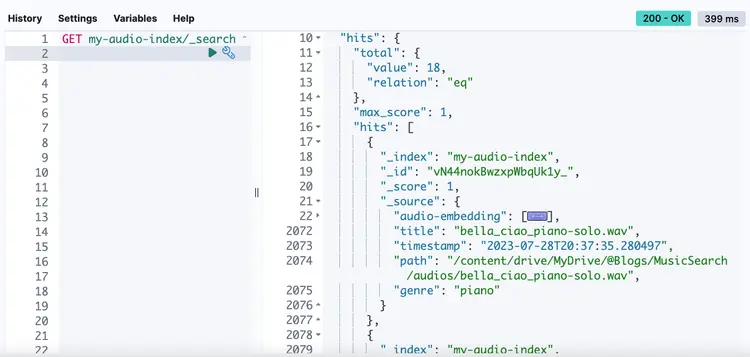
5、按音乐搜索
现在,你可以使用生成的嵌入执行向量相似性搜索。当你向系统提供输入歌曲时,它会将歌曲转换为嵌入,在数据库中搜索类似的嵌入,并返回具有相似特征的歌曲。
# Define a function to query audio vector in Elasticsearch
def query_audio_vector(es, emb, field_key, index_name):
# Initialize the query structure
query = {
"bool": {
"filter": [{
"exists": {
"field": field_key
}
}]
}
}
# KNN search parameters
# field is the name of the field to perform the search on
# k is the number of nearest neighbors to find
# num_candidates is the number of candidates to consider (more means slower but potentially more accurate results)
# query_vector is the vector to find nearest neighbors for
# boost is the multiplier for scores (higher means this match is considered more important)
knn = {
"field": field_key,
"k": 2,
"num_candidates": 100,
"query_vector": emb,
"boost": 100
}
# The fields to retrieve from the matching documents
fields = ["title", "path", "genre", "body_content", "url"]
# The name of the index to search
index = index_name
# Perform the search
resp = es.search(index=index,
query=query,
knn=knn,
fields=fields,
size=5,
source=False)
# Return the search results
return resp
让我们从有趣的部分开始吧!
5.1 选择要搜索的音乐
在下面的代码中,我们直接从 GitHub 音频目录中选择音乐,并使用音频音乐在 Google Colab 中播放结果。
# Import necessary modules for audio display from IPython
from IPython.display import Audio, display
# Provide the URL of the audio file
my_audio = "/content/drive/MyDrive/@Blogs/MusicSearch/audios/bella_ciao_humming.wav"
# Display the audio file in the notebook
Audio(my_audio)
你可以点击这里播放音乐。
5.2 搜索音乐
现在,让我们运行代码在 Elasticsearch 中搜索音乐“my_audio”。我们将仅使用音频文件进行搜索。
# Generate the embedding vector from the provided audio file
emb = get_embedding(audio_file)
# Query the Elasticsearch instance 'es' with the embedding vector 'emb', field key 'audio-embedding',
# and index name 'my-audio-index'
resp = query_audio_vector (es, emb.tolist(), "audio-embedding", "my-audio-index" )
Elasticsearch 将返回所有与您的关键歌曲类似的音乐:
{
'total': {
'value': 18,
'relation': 'eq'
},
'max_score': 100.0,
'hits': [
{
'_index': 'my-audio-index',
'_id': 'tt44nokBwzxpWbqUfVwN',
'_score': 100.0,
'fields': {
'path': ['/content/drive/MyDrive/@Blogs/MusicSearch/audios/bella_ciao_humming.wav'],
'genre': ['humming'],
'title': ['bella_ciao_humming.wav']
}
},
{
'_index': 'my-audio-index',
'_id': 'u944nokBwzxpWbqUj1zy',
'_score': 86.1148,
'fields': {
'path': ['/content/drive/MyDrive/@Blogs/MusicSearch/audios/bella_ciao_opera-singer.wav'],
'genre': ['opera'],
'title': ['bella_ciao_opera-singer.wav']
}
},
{
'_index': 'my-audio-index',
'_id': 'vt44nokBwzxpWbqUm1xK',
'_score': 0.0,
'fields': {
'path': ['/content/drive/MyDrive/@Blogs/MusicSearch/audios/bella_ciao_tribal-drums-and-flute.wav'],
'genre': ['generic'],
'title': ['bella_ciao_tribal-drums-and-flute.wav']
}
},
{
'_index': 'my-audio-index',
'_id': 'uN44nokBwzxpWbqUhFye',
'_score': 0.0,
'fields': {
'path': ['/content/drive/MyDrive/@Blogs/MusicSearch/audios/bella_ciao_electronic-synth-lead.wav'],
'genre': ['generic'],
'title': ['bella_ciao_electronic-synth-lead.wav']
}
},
{
'_index': 'my-audio-index',
'_id': 'wN44nokBwzxpWbqUpFyT',
'_score': 0.0,
'fields': {
'path': ['/content/drive/MyDrive/@Blogs/MusicSearch/audios/a-cappella-chorus.wav'],
'genre': ['generic'],
'title': ['a-cappella-chorus.wav']
}
}
]
}
一些有助于播放结果的代码:
NUM_MUSIC = 5 # example value
for i in range(NUM_MUSIC):
path = resp['hits']['hits'][i]['fields']['path'][0]
print(path)
content/drive/MyDrive/@Blogs/MusicSearch/audios/bella_ciao_humming.wav /content/drive/MyDrive/@Blogs/MusicSearch/audios/bella_ciao_humming.wav /content/drive/MyDrive/@Blogs/MusicSearch/audios/bella_ciao_a-cappella-chorus.wav /content/drive/MyDrive/@Blogs/MusicSearch/audios/bella_ciao_electronic-synth-lead.wav /content/drive/MyDrive/@Blogs/MusicSearch/audios/bella_ciao_guitar-solo.wav
Audio("/content/drive/MyDrive/@Blogs/MusicSearch/audios/bella_ciao_a-cappella-chorus.wav")
现在,您可以单击“播放”来检查结果。
5.3 分析结果
那么,我可以在生产环境中部署此代码并销售我的应用程序吗?不,作为概率模型,概率听觉神经网络 (PANN) 和任何其他机器学习模型都需要增加数据量和额外的微调才能有效地应用于实际场景。
可视化与我们的 18 首歌曲样本相关的嵌入的图表可以证明这一点,这可能会导致 kNN 方法出现误报。然而,未来的数据工程师仍面临一个显著的挑战:通过哼唱确定查询的最佳模型。这代表了机器学习和听觉认知的一个迷人交集,需要严谨的研究和创新的问题解决。
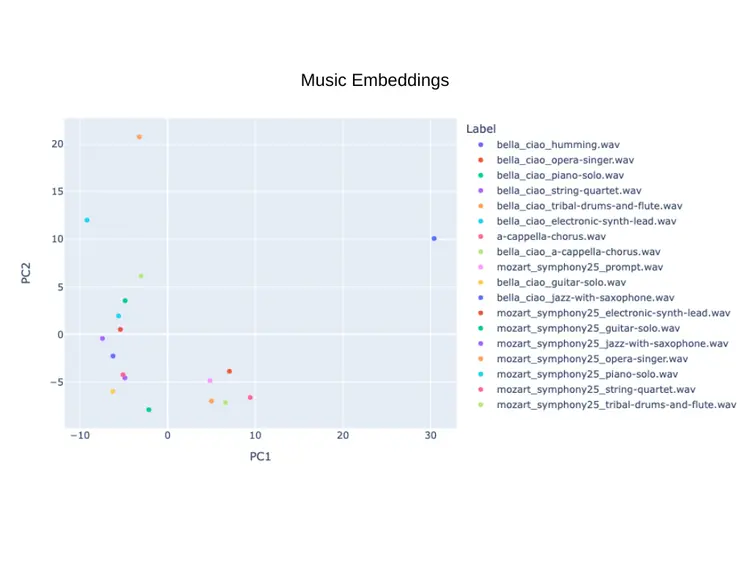
5.4 使用 UI 改进 POC(可选)
经过一点修改,我将整个代码复制并粘贴到 Streamlit。Streamlit 是一个 Python 库,可简化为数据科学和机器学习项目创建交互式 Web 应用程序的过程。它允许新手轻松地将数据脚本转换为可共享的 Web 应用程序,而无需广泛的 Web 开发知识。
结果就是这个应用程序:
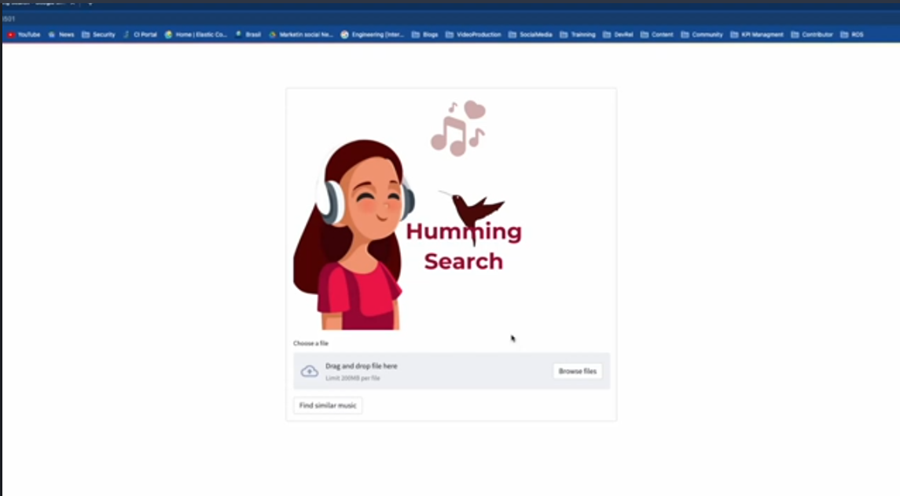
我们已经成功地使用 Python 中的 Elasticsearch 向量实现了一个音乐搜索系统。 这是音频搜索领域的起点,并且可以通过利用这种架构方法激发更多创新概念。 通过改变模型,可以开发不同的应用程序。 此外,将推理移植到 Elasticsearch 可能会提高性能。 访问 Elastic 的机器学习页面以了解更多信息。
这表明这项技术对于文本以外的各种搜索应用程序具有巨大的潜力和适应性。
原文链接:Searching by music: Leveraging vector search for audio information retrieval
汇智网翻译整理,转载请标明出处