大模型微调改善SQL生成
在使用Mistral 7B LLM 执行 SQL 生成任务时,我遇到了挑战,尤其是在处理公司的数据库时。这些模型通常难以生成准确的 SQL 查询,即使在上下文中提供了数据库架构和表关系。为了应对这一挑战,使用 QLoRA 在针对特定数据库架构定制的自定义数据集上微调 7B 模型是一种有效的方法。
在本文中,我将引导你完成微调 7B 模型以更有效地处理 SQL 生成任务的过程,以及如何将微调后的模型集成到基于 LangChain 的应用程序中以实现实时数据库交互。
1、概述
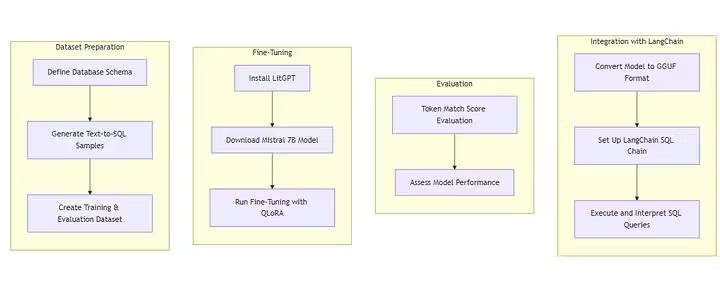
在深入研究细节之前,让我们概述一下我们将在本指南中遵循的关键步骤:
- 根据数据库架构准备自定义数据集。
- 使用 QLoRA 技术微调 7B 模型。
- 评估微调模型的性能。
- 将模型集成到 LangChain 应用程序中以进行基于 SQL 的数据库交互。
通过遵循本指南,你将能够使用经过微调的 Mistral 7B 模型为 SQL 数据库构建问答应用程序,该模型针对根据你的特定数据库架构生成 SQL 查询进行了优化。
2、准备你的自定义数据集
要有效地微调模型,你需要一个能够反映数据库结构的高质量数据集。
让我们考虑一个包含3个表的简单客户管理数据库:
CREATE TABLE customer (
customer_key INT PRIMARY KEY,
source VARCHAR(50),
full_name VARCHAR(100),
created_date DATETIME,
updated_date DATETIME,
gender VARCHAR(10),
dateofbirth DATE
);
CREATE TABLE address (
address_key INT PRIMARY KEY,
customer_key INT,
street_address VARCHAR(200),
city VARCHAR(100),
state VARCHAR(50),
postal_code VARCHAR(20),
country VARCHAR(50),
is_primary BOOLEAN,
created_date DATETIME,
updated_date DATETIME,
FOREIGN KEY (customer_key) REFERENCES customer(customer_key)
);
CREATE TABLE contact (
contact_key INT PRIMARY KEY,
customer_key INT,
email VARCHAR(100),
phone VARCHAR(20),
created_date DATETIME,
updated_date DATETIME,
FOREIGN KEY (customer_key) REFERENCES customer(customer_key)
);
2.1 创建文本到 SQL 样本
要生成用于微调的数据集,请使用 Claude Sonnet 或其他 LLM 创建文本到 SQL 的样本。以下是可用于指导模型创建 SQL 查询的提示格式:
Sample Format:
{
"instruction": "I want you to act as a SQL terminal in front of an example database. You need only to return the SQL command to me. Below is an instruction that describes a task. Write a response that appropriately completes the request.
## Instruction:
[Database description]
",
"input": "### Input:
[Natural language question]
### Response:",
"output": "[Corresponding SQL query]"
}
Sample Data Point:
{
"instruction": "You are a powerful text-to-SQL model. Your task is to generate SQL queries based on the following schema for a customer database:\n\nCREATE TABLE customer (\n customer_key INT PRIMARY KEY,\n source VARCHAR(50),\n full_name VARCHAR(100),\n created_date DATETIME,\n updated_date DATETIME,\n gender VARCHAR(10),\n dateofbirth DATE\n);\n\nCREATE TABLE address (\n address_key INT PRIMARY KEY,\n customer_key INT,\n street_address VARCHAR(200),\n city VARCHAR(100),\n state VARCHAR(50),\n postal_code VARCHAR(20),\n country VARCHAR(50),\n is_primary BOOLEAN,\n created_date DATETIME,\n updated_date DATETIME,\n FOREIGN KEY (customer_key) REFERENCES customer(customer_key)\n);\n\nCREATE TABLE contact (\n contact_key INT PRIMARY KEY,\n customer_key INT,\n email VARCHAR(100),\n phone VARCHAR(20),\n created_date DATETIME,\n updated_date DATETIME,\n FOREIGN KEY (customer_key) REFERENCES customer(customer_key)\n);",
"input": "List the full names of customers who have both an email and a phone number.",
"output": "SELECT DISTINCT c.full_name\nFROM customer c\nJOIN contact ct ON c.customer_key = ct.customer_key\nWHERE ct.email IS NOT NULL AND ct.phone IS NOT NULL;"
}
The database contains three tables: customer, address, and contact.
Table 'customer' has columns:
customer_key (INT, primary key)
source (VARCHAR(50))
full_name (VARCHAR(100))
created_date (DATETIME)
updated_date (DATETIME)
gender (VARCHAR(10))
dateofbirth (DATE)
Table 'address' has columns:
address_key (INT, primary key)
customer_key (INT, foreign key referencing customer(customer_key))
street_address (VARCHAR(200))
city (VARCHAR(100))
state (VARCHAR(50))
postal_code (VARCHAR(20))
country (VARCHAR(50))
is_primary (BOOLEAN)
created_date (DATETIME)
updated_date (DATETIME)
Table 'contact' has columns:
contact_key (INT, primary key)
customer_key (INT, foreign key referencing customer(customer_key))
email (VARCHAR(100))
phone (VARCHAR(20))
created_date (DATETIME)
updated_date (DATETIME)
Please generate 100 samples in JSON file based on the provided database schema and example. For each sample, ensure that:
Instruction: include only the necessary table definitions in the instruction based on the SQL query in the output
Input: Contains a natural language question about the data.
Output: Provides the corresponding SQL query that answers the question.
The questions should cover topics such as data analysis, aggregation, address searches, customer searches, contact searches, and reporting.
重复此过程,生成约 200 到 500 个样本,涵盖各种 SQL 任务,包括客户查询、地址查找和数据聚合。这些样本应以 JSON 格式保存,并分别拆分为 train.json 和 eval.json 以进行训练和评估。
数据集示例:
[
{
"instruction": "You are a powerful text-to-SQL model. Your job is to answer questions about the customer database based on the provided SCHEMA.\nYou must output the SQL query that answers the question. SCHEMA:\nCREATE TABLE customer (\n customer_key INT PRIMARY KEY,\n full_name VARCHAR(100),\n dateofbirth DATE\n);",
"input": "List the full names of all customers born after January 1, 1990.",
"output": "SELECT full_name FROM customer WHERE dateofbirth > '1990-01-01';"
},
{
"instruction": "You are a powerful text-to-SQL model. Your job is to answer questions about the address data based on the provided SCHEMA.\nYou must output the SQL query that answers the question. SCHEMA:\nCREATE TABLE address (\n address_key INT PRIMARY KEY,\n customer_key INT,\n city VARCHAR(100)\n);",
"input": "Find all unique cities where customers reside.",
"output": "SELECT DISTINCT city FROM address;"
},
{
"instruction": "You are a powerful text-to-SQL model. Your job is to answer questions about customer emails based on the provided SCHEMA.\nYou must output the SQL query that answers the question. SCHEMA:\nCREATE TABLE customer (\n customer_key INT PRIMARY KEY\n);\n\nCREATE TABLE contact (\n contact_key INT PRIMARY KEY,\n customer_key INT,\n email VARCHAR(100)\n);",
"input": "Find customers who have not provided an email address.",
"output": "SELECT c.customer_key FROM customer c LEFT JOIN contact ct ON c.customer_key = ct.customer_key WHERE ct.email IS NULL;"
},
{
"instruction": "You are a powerful text-to-SQL model. Your job is to answer questions about customers without addresses based on the provided SCHEMA.\nYou must output the SQL query that answers the question. SCHEMA:\nCREATE TABLE customer (\n customer_key INT PRIMARY KEY\n);\n\nCREATE TABLE address (\n address_key INT PRIMARY KEY,\n customer_key INT\n);",
"input": "List customer keys of customers who have no address on file.",
"output": "SELECT c.customer_key FROM customer c LEFT JOIN address a ON c.customer_key = a.customer_key WHERE a.customer_key IS NULL;"
},
...
]
3、微调模型
数据集准备就绪后,下一步是使用 QLoRA 微调Mistral 7B 模型。有几种方法可用于微调模型:
- Hugging Face TRL 和 SFTTrainer
- DB-GPT-Hub:在公共文本到 SQL 数据集(如 Spider)上微调模型的不错选择。
- LitGPT:用于快速微调、预训练和部署 LLM 的轻量级高效框架。
对于我们的用例,我们将使用 LitGPT 在客户数据库架构上微调我们的 7B 模型。
安装 LitGPT:
pip install litgpt
下载模型权重:
litgpt download mistralai/Mistral-7B-Instruct-v0.3 --access_token=xxxxxx
使用 4 位量化运行微调过程:
litgpt finetune_lora \
checkpoints/mistralai/Mistral-7B-Instruct-v0.3 \
--data JSON \
--data.json_path train.json \
--out_dir finetuned \
--precision bf16-true \
--quantize "bnb.nf4" \
--lora_r 8 \
--lora_alpha 16 \
--lora_dropout 0.05 \
--train.global_batch_size 4 \
--train.micro_batch_size 1 \
--train.max_steps 1000 \
--train.save_interval 200 \
--eval.interval 50 \
--train.lr_warmup_steps 100 \
--train.max_seq_length 2048 \
--optimizer.learning_rate 2e-4 \
--optimizer.weight_decay 0.01 \
--optimizer.betas 0.9 \
--data.val_split_fraction 0.1
有关更详细的说明,请参阅官方 LitGPT 文档。
4、评估微调模型
微调后,评估模型的性能至关重要。我们将使用 Token Match 分数指标来实现此目的:
使用前面创建的 evolve.json 文件,其中包含示例 SQL 查询。
将合并后的权重从 LitGPT ( /finetuned/final/lit_model.pth
) 转换为 Hugging Face Transformers 格式。
litgpt convert_from_litgpt finetuned/final out/hf-mistral-7b/converted
运行评估脚本:
import json
import torch
from transformers import AutoModelForCausalLM, AutoTokenizer
from huggingface_hub import login
import re
import sqlparse
from sklearn.metrics import accuracy_score
login(token="XXXXXXX")
# Load the fine-tuned LoRA model
model_path = "out/hf-mistral-7b/converted"
tokenizer = AutoTokenizer.from_pretrained("mistralai/Mistral-7B-Instruct-v0.3")
state_dict = torch.load(f"{model_path}/model.pth")
model = AutoModelForCausalLM.from_pretrained("mistralai/Mistral-7B-Instruct-v0.3", state_dict=state_dict)
model.to("cuda:0")
# Load the evaluation dataset
with open("evaluate.json", "r") as f:
eval_data = json.load(f)
def normalize_sql(sql):
# Remove comments
sql = re.sub(r'--.*$', '', sql, flags=re.MULTILINE)
sql = ' '.join(sql.split())
parsed = sqlparse.parse(sql)[0]
return str(parsed).lower()
def exact_match_score(prediction, reference):
return normalize_sql(prediction) == normalize_sql(reference)
def token_match_score(prediction, reference):
pred_tokens = set(re.findall(r'\b\w+\b', normalize_sql(prediction)))
ref_tokens = set(re.findall(r'\b\w+\b', normalize_sql(reference)))
return len(pred_tokens.intersection(ref_tokens)) / len(ref_tokens) if ref_tokens else 0
def evaluate_model(model, tokenizer, eval_data):
exact_matches = []
token_match_scores = []
for item in eval_data:
instruction = item["instruction"]
input_question = item.get("input", "")
expected_output = item["output"]
messages = [
{"role": "system", "content": instruction},
{"role": "user", "content": input_question},
]
encodeds = tokenizer.apply_chat_template(messages, return_tensors="pt", padding_side='left')
model_inputs = encodeds.to("cuda:0")
outputs = model.generate(model_inputs, max_new_tokens=150)
decoded = tokenizer.batch_decode(outputs[:, model_inputs.shape[1]:], skip_special_tokens=True)
sql_query = decoded[0]
print(f"Instruction: {instruction}")
print(f"Input Question: {input_question}")
print(f"Expected Output: {expected_output}")
print(f"Generated Output: {sql_query}")
print("=" * 50)
# Compute metrics
exact_matches.append(exact_match_score(sql_query, expected_output))
token_match_scores.append(token_match_score(sql_query, expected_output))
avg_token_match_score = sum(token_match_scores) / len(token_match_scores)
print(f"Average Token Match Score: {avg_token_match_score:.4f}")
evaluate_model(model, tokenizer, eval_data)
此评估方法可以让你更好地了解模型在文本到 SQL 任务上的性能。
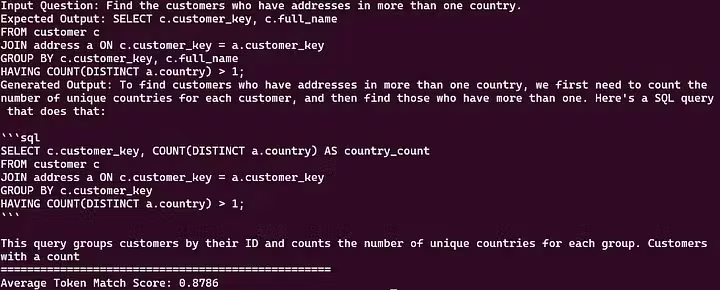
如你所见,0.8786 的得分对于文本到 SQL 模型来说相当不错。
5、使用 LangChain 构建数据库交互 RAG
成功微调和评估模型后,我们现在可以将其集成到 LangChain 应用程序中以构建数据库交互应用程序。
首先安装 LangChain 和 llama.cpp:
set FORCE_CMAKE=1 && set CMAKE_ARGS=-DGGML_CUDA=on && pip install llama-cpp-python --extra-index-url https://abetlen.github.io/llama-cpp-python/whl/124
将 Hugging Face 模型转换为 GGUF 格式:
python convert_hf_to_gguf.py /path/to/hf-model --outfile custom-mistral-7b.gguf --outtype f16
量化模型以减小尺寸(可选):
./llama-quantize ./custom-mistral-7b.gguf ./custom-mistral-7b-Q5_K_M.gguf Q5_K_M
我建议使用 Q5_K_M,因为它可以保留模型的大部分性能。或者,如果你想节省一些内存,您可以选择 Q4_K_M。
安装 LangChain 和所需的库。
实现 LangChain SQL 链:
import re
from langchain.sql_database import SQLDatabase
from langchain_community.llms import LlamaCpp
from langchain_community.utilities import SQLDatabase
from langchain.chains import create_sql_query_chain
from langchain_core.runnables import RunnablePassthrough
from langchain_core.prompts.chat import ChatPromptTemplate
# Initialize the LlamaCpp language model with specified parameters
llm = LlamaCpp(
model_path="./custom-mistral-7b-Q5_K_M.gguf", # Path to the model file
max_tokens=2048, # Maximum number of tokens in the response
n_ctx=6144, # Context size
verbose=True,
temperature=0,
)
# Define the dataset and SQLAlchemy connection URL
dataset = "customer"
sqlalchemy_url = (
f"postgresql://db_user:db_pass@db_host:5432" # Replace with actual credentials and host
)
# Initialize the SQLDatabase object with specified schema and tables
db = SQLDatabase.from_uri(
sqlalchemy_url,
schema=dataset,
include_tables=['customer', 'address', 'contact'] # Tables to include in the database
)
# Create the SQL query generation chain using the language model and database
gen_query = create_sql_query_chain(llm, db)
# Convert datetime objects in strings to a specific format
def convert_dates(obj):
response_str = re.sub(
r'datetime\.date\((\d+),\s*(\d+),\s*(\d+)\)',
r"'\1-\2-\3'",
obj
)
response_str = re.sub(
r'datetime\.datetime\((\d+),\s*(\d+),\s*(\d+),\s*(\d+),\s*(\d+),\s*(\d+)(?:,\s*(\d+))?\)',
r"'\1-\2-\3 \4:\5:\6.\7'",
response_str
)
return response_str
# Route the SQL query based on its content
def route(sql_query: str):
logging.info(f"Routing query: {sql_query}") # Log the query for debugging
if sql_query.get("query") == "I don't know":
logging.warning("Unknown query detected.") # Warn if the query is unknown
return sql_query # Return the original query
else:
return db_opt_chain # Route to the database operation chain
# Handle cases where the agent responds with "I don't know"
def handle_dont_know(result):
if isinstance(result, dict) and result.get("query") == "I don't know":
return "I can only provide information related to our customer data." # Custom response
return result # Return the original result if not "I don't know"
# Custom function to execute the SQL query and process the result
def custom_execute_query_runnable(result: dict) -> dict:
return {
**result, # Include existing result data
'result': convert_dates(
db.run_no_throw(command=result["query"], include_columns=True) # Execute the query without throwing exceptions
)
}
# Template for generating the final natural language response
template = """Based on the table schema below, question, sql query, and sql response, write a natural language response:
{schema}
Question: {question}
SQL Query: {query}
SQL Response: {response}"""
# Define the prompt for generating the response
prompt_response = ChatPromptTemplate.from_template(template)
# Define the database operation chain with the custom execution function and response prompt
db_opt_chain = (
RunnableLambda(custom_execute_query_runnable) # Execute the query
| answer_prompt # Generate the answer based on the query result
| llm # Use the language model to format the answer
)
# Combine all components into the full execution chain
full_chain = (
RunnablePassthrough().assign(query=gen_query) # Pass through the query generation
| RunnableLambda(route) # Route the query appropriately
| RunnableLambda(handle_dont_know) # Handle "I don't know" responses
| StrOutputParser() # Parse the final output as a string
)
# Example user question to invoke the chain
user_question = 'Get the minimum and maximum age of customers'
full_chain.invoke({"question": user_question}) # Execute the chain with the user question
注意:本指南中提供的示例代码仅供参考。你需要调整代码以适合你的特定用例,例如修改数据库连接字符串、架构定义或根据你的数据和基础架构要求调整模型参数。
此实现会创建一个链,该链使用经过微调的 LLM 生成 SQL,针对数据库执行查询,然后再次使用 LLM 解释和总结结果。
6、结束语
通过使用针对特定数据库架构定制的自定义数据集对 7B LLM 进行微调,您可以大大增强其 SQL 生成功能。与 LangChain 结合使用时,即使使用较小的语言模型,您也可以构建用于数据库交互的问答应用程序。
请记住,微调模型的有效性取决于训练数据集的质量和多样性。随着时间的推移,不断完善数据集和微调过程将带来更好的结果。
原文链接:Improving Text-to-SQL with a Fine-Tuned 7B LLM for DB Interactions
汇智网翻译整理,转载请标明出处