Cursor开发提速:上下文压缩
Cursor 项目通常遵循类似的模式。你会迅速取得进展,然后撞上一堵砖墙,一切都似乎变得糟糕且耗时。
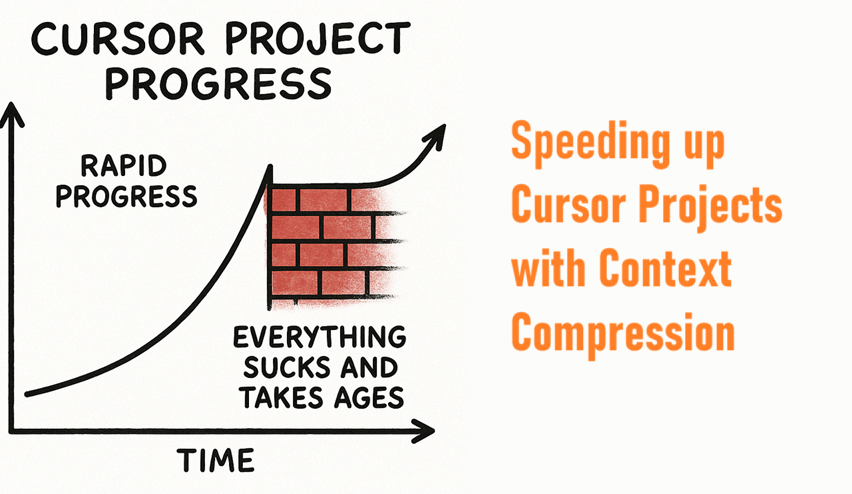
Cursor 项目通常遵循类似的模式。你会迅速取得进展,然后撞上一堵砖墙,一切都似乎变得糟糕且耗时。
我经常收到的一个常见问题是某种形式的:
“Cursor 在大型项目中的表现如何?你对这些更大的项目有什么技巧和窍门吗?”
所以这将是关于处理更大项目的系列文章的第一部分。
对于这篇文章,我们将专注于上下文处理,并且我们不会简单地将所有内容塞进 Gemini 2.5,因为这在 Cursor 中不起作用。
1、大型项目中最大的痛点是……大量的代码
因此,我们的一个关键指导原则是:“只发送模型真正需要的内容,并以它仍能理解的最简洁的形式。”
尽管 Cursor 大肆宣传它可以理解整个代码库,但现实是目前它并不能做到这一点,这一点在处理较大的任务时会通过几个明显的迹象体现出来。你的 grep 调用和搜索工具调用开始激增。
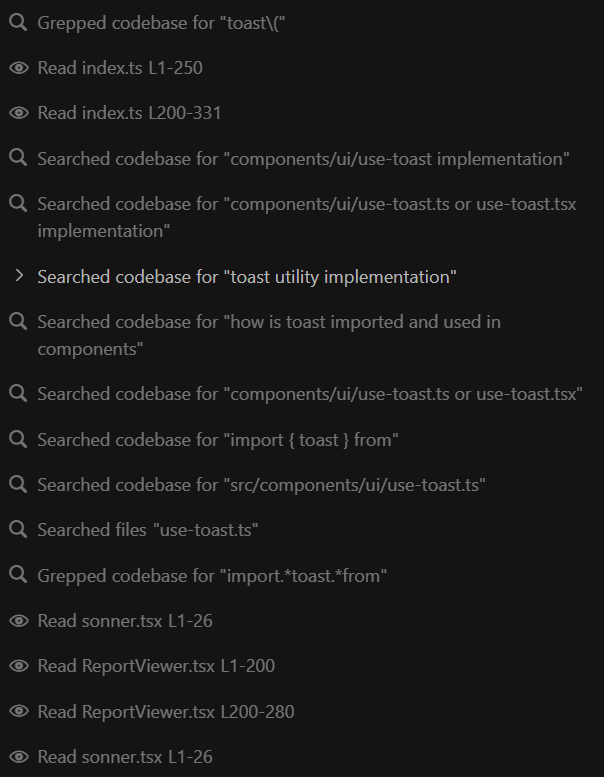
理论上能够搜索代码库和实际拥有整个代码库并在内存中保持上下文是完全不同的事情。
换句话说,如果 Cursor 实际上能够完美记住你的代码库,它就不需要花费这么多时间去搜索了。
所有这些都代表了在更大项目中日益严重的问题。文件越长,搜索越多,等待越多,迭代周期越长,高级请求越多。
没有什么比数据库模式文件更能体现这一点了。
随着你的模式增长(以及相关的子组件)到数千行,每增加一个表和调用都会显著变慢。
然后所有的 API 调用开始变慢。接着你开始达到工具调用的 25 次限制,这一切开始耗费金钱。
那么我们该怎么办呢?直接跳到 Gemini 2.5 吗?不。
在撰写本文并尝试解决自己的问题时,我尝试了几种方法。
- 我尝试了 Jinni 和其关联的 MCP 服务器。据说这可以更快地自由访问代码库,并且意味着 Cursor 检索它时不需要工具调用。听起来很棒,但在实践中它总是限制上下文长度,因此失败了。
- 我尝试了 Taskmaster AI,它旨在帮助处理更大的项目并将事情分解为待办事项列表。它确实有效,但同样非常缓慢。在它搜索完一切之前,我本可以用手动编写一些组件的代码。
基本上我发现这些产品只是试图解决大海捞针的问题,并将所有内容塞入上下文中,这感觉并不正确。
2、我们需要一个更快的指针系统
我的目标是重新设计一个更快的指针系统来处理模式。
我们的目标本质上是为 Cursor 提供一个更干净的交互版本的模式,而不是整个东西。
一个正常的 Drizzle 模式看起来像这样:
// 报告模块系统表
export const reportModules = createTable(
"report_module",
(d) => ({
id: d.varchar({ length: 255 }).primaryKey(),
name: d.varchar({ length: 255 }).notNull(),
description: d.text(),
defaultPromptText: d.text().notNull(),
isTemplate: d.boolean().default(false),
templateId: d.varchar({ length: 255 }),
contextQueries: d.json(), // 存储预定义查询及其 UI 表示
displayOrder: d.int().default(0),
createdAt: d
.timestamp()
.default(sqlCURRENT_TIMESTAMP)
.notNull(),
updatedAt: d.timestamp().onUpdateNow(),
}),
(t) => [
index("is_template_idx").on(t.isTemplate),
],
);
// Legend: PK=primary key, FK→table.column, ts=timestamp
T exception:
id int PK, lineout txt, game v255, status v20=to_review, createdAt ts
T image:
id int PK, exceptionId int FK→exception.id, url txt
T game:
id v255 PK, name v255, team1 v255, team2 v255, status v20
T lineout:
id v255 PK, gameId v255 FK→game.id, team v255, throwDist v255, outcome v255, …
T event:
id v255 PK, lineoutId v255 FK→lineout.id, type v100, t secs, cam1frame int, …
上面的模式是我制作的“压缩元模式”——基本上剥离了模式的核心并给了模型一个高层次的概述。
为什么它有效
- 每行少于 20 个标记;模型很容易解析“
T 名称:
然后用逗号分隔的列”的模式。 - 类型减少到 2-3 个字母(
v255
、int
、txt
、ts
)——模型只需要序数比较(字符串与数字),其余的可以推断。 - FK 箭头在两个标记中明确关系(
FK→
)。
对于完整的模式(约 1900 个标记的原始 TypeScript),这减少到约 220 个标记——节省了 9 倍。
现在相信我,我以前并不是特别热衷于优化上下文标记,但坐在那里等待大型项目完成任何事情会让你成为一个上下文窗口保守派。
在我开始压缩模式后,我立即发现它指向了它需要知道的内容。
我还添加了一个顶部标题:
Interpret columns as (name type [=default]) and follow FK arrows for relations;
assume VARCHAR unless another type code is given; ts means TIMESTAMP; txt means TEXT.
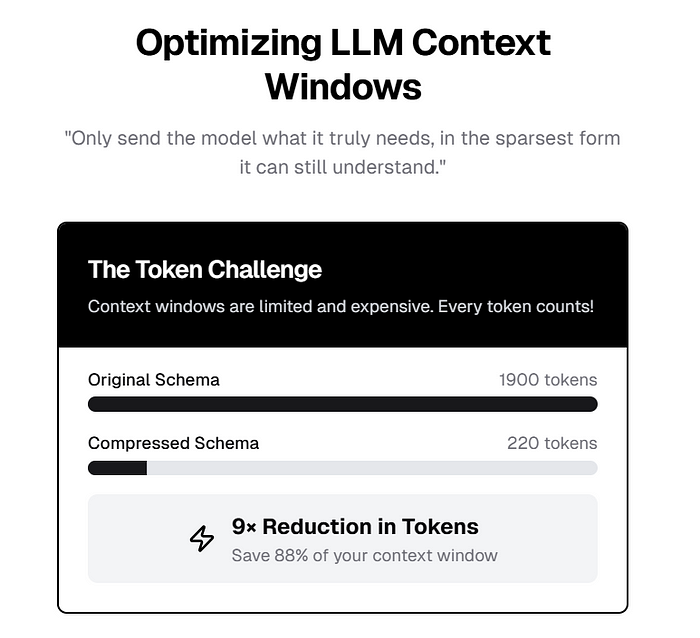
这只是针对一个文件!一旦你开始压缩,你可以开始优化各种各样的东西
但我们还可以做得更好。受 Cursor 团队的启发,我意识到解锁性能的关键是构建工具管道,这为你提供了一个全新的解锁。
与 LLMs 工作的乐趣之一就是你可以让它为你创建管道。
首先在 tools/compress.ts 或者你语言等效的地方创建一个类似的文件。我粘贴了这个并要求它为 Python 脚本生成一个,它一次就完成了。
// compress-schema.ts - 将 Drizzle createTable() 声明转换为每表一行的 DSL
// -----------------------------------------------------------------------------
// 使用示例
// pnpm schema:compress # 默认扫描 src/**
// pnpm schema:compress --root src/server/db # 指向子文件夹
// pnpm schema:compress --only games,lineouts # 仅限特定表
// -----------------------------------------------------------------------------
import { Command } from "commander";
import fg from "fast-glob";
import { Project, SyntaxKind, Node, PropertyAssignment } from "ts-morph";
import clipboard from "clipboardy";
import fs from "fs";
import path from "path";
/** 将 Drizzle 列构造器名称和可选长度映射为 2-3 个字符的令牌。 */
function mapType(type: string, len?: string): string {
const n = len?.replace(/\D/g, "");
switch (type) {
case "varchar": return `v${n || 255}`;
case "text": return "txt";
case "int": return "int";
case "double": return "dbl";
case "boolean": return "bool";
case "timestamp": return "ts";
case "json": return "json";
default: return type;
}
}
function isPrimaryKey(callText: string) {
return /\.primaryKey\(/.test(callText);
}
const cli = new Command()
.option("--root <dir>", "要扫描的文件夹 (默认: src)", "src")
.option("--only <tables>", "逗号分隔的允许列表(区分大小写)")
.parse(process.argv)
.opts();
const allow = cli.only ? new Set<string>(cli.only.split(",")) : null;
const project = new Project({ tsConfigFilePath: "tsconfig.json" });
fg.sync(`${cli.root}/**/*.ts`).forEach((f) => project.addSourceFileAtPath(f));
const outLines: string[] = [];
project.getSourceFiles().forEach((sf) => {
sf.getDescendantsOfKind(SyntaxKind.CallExpression)
.filter((ce) => ce.getExpression().getText().startsWith("createTable"))
.forEach((ce) => {
const [nameNode, columnsFn, indexesFn] = ce.getArguments();
const tblName = nameNode.asKindOrThrow(SyntaxKind.StringLiteral).getLiteralText();
if (allow && !allow.has(tblName)) return;
const arrow = columnsFn.asKindOrThrow(SyntaxKind.ArrowFunction);
let body = arrow.getBody();
if (Node.isParenthesizedExpression(body)) body = body.getExpression();
const obj = body.asKindOrThrow(SyntaxKind.ObjectLiteralExpression);
const colDefs: string[] = [];
obj.getProperties().forEach((prop) => {
if (!Node.isPropertyAssignment(prop)) return;
const p = prop as PropertyAssignment;
const colName = p.getName();
const initText = p.getInitializer()?.getText() ?? "";
const typeMatch = initText.match(/\.([a-zA-Z]+)\(/);
const lenMatch = initText.match(/length:\s*(\d+)/);
const mapped = mapType(typeMatch?.[1] ?? "unknown", lenMatch?.[1]);
const pk = isPrimaryKey(initText) ? " PK" : "";
colDefs.push(`${colName} ${mapped}${pk}`);
});
if (indexesFn) {
const arrFn = indexesFn.asKindOrThrow(SyntaxKind.ArrowFunction);
let idxBody = arrFn.getBody();
if (Node.isParenthesizedExpression(idxBody)) idxBody = idxBody.getExpression();
if (Node.isArrayLiteralExpression(idxBody)) {
idxBody.getElements().forEach((el) => {
if (!Node.isCallExpression(el)) return;
if (!/foreignKey/.test(el.getExpression().getText())) return;
const cfgObj = el.getArguments()[0]?.asKind(SyntaxKind.ObjectLiteralExpression);
if (!cfgObj) return;
const colsArr = cfgObj.getProperty("columns")?.getFirstDescendantByKind(SyntaxKind.ArrayLiteralExpression);
const fcolsArr = cfgObj.getProperty("foreignColumns")?.getFirstDescendantByKind(SyntaxKind.ArrayLiteralExpression);
const colTxt = colsArr?.getElements()[0]?.getText().replace(/^.+\./, "");
const fcolTxt = fcolsArr?.getElements()[0]?.getText().replace(/^.+\./, "");
const ftabTxt = fcolsArr?.getElements()[0]?.getText().split(".")[0];
if (colTxt && ftabTxt && fcolTxt) colDefs.push(`${colTxt} FK→${ftabTxt}.${fcolTxt}`);
});
}
}
outLines.push(`T ${tblName}: ${colDefs.join(", ")}`);
});
});
const decodeHeader = `// 压缩版 Drizzle 模式 DSL
// 说明:PK = 主键,FK→ = 外键,ts = 时间戳,txt = 文本,vXXX = VARCHAR(XXX),dbl = 双精度,bool = 布尔值
`;
const finalDslWithHeader = `${decodeHeader}\n${outLines.join("\n")}`;
console.log(finalDslWithHeader);
try {
clipboard.writeSync(finalDslWithHeader);
console.error("📋 已复制到剪贴板");
} catch { }
try {
const dirPath = path.resolve("output");
const filePath = path.join(dirPath, "compress");
if (!fs.existsSync(dirPath)) fs.mkdirSync(dirPath);
fs.writeFileSync(filePath, finalDslWithHeader);
console.error("📄 已保存到 ./output/compress");
} catch (err) {
console.error("❌ 保存输出文件失败", err);
}
在你完成上述操作后,修改你的 package.json 文件:
"scripts": {
"schema:compress": "tsx tools/compress-schema.ts",
"schema:compress:games": "pnpm schema:compress --only games,lineouts",
…
}
注意,在上面的内容中,我将 games 和 lineouts 作为一个命令,因为我经常过滤这些表。你可以让你的 LLM 调整这个命令,或者完全删除它。
然后我们需要安装一些东西:
pnpm add -D commander fast-glob ts-morph clipboardy
现在你已经准备好运行命令了:
pnpm schema:compress --root src/server/db
运行上述命令后,你应该会在 output 文件夹中得到一个文件。
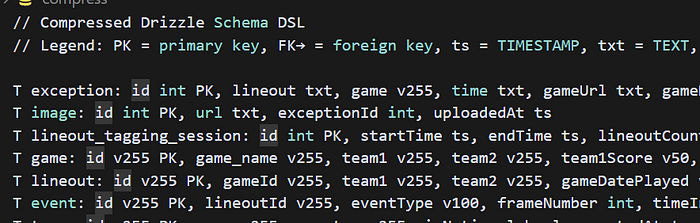
我们得到了一个很好的压缩模式文件。
然后你可以直接在 Cursor 聊天中引用这个文件作为上下文,并记住在文件顶部添加指令,以便模型知道如何解码它。
这里是你可以让模型执行的一些策略。
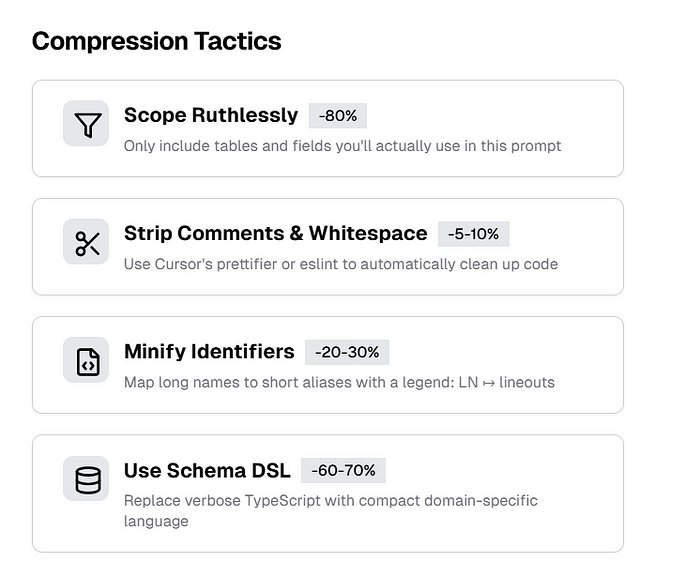
基本上,只要能找到任何方法来减少上下文即可。
3、新的工作流程
如果我更新了模式,我已经设置了 pnpm 命令,可以在 pnpm db:push 后自动生成这个文件,这样我就始终拥有一个最新且更新的压缩模式。
接下来,我整理了我的文件结构,因为我已经厌倦了通过 grep 查找文件并弄丢它们的位置。
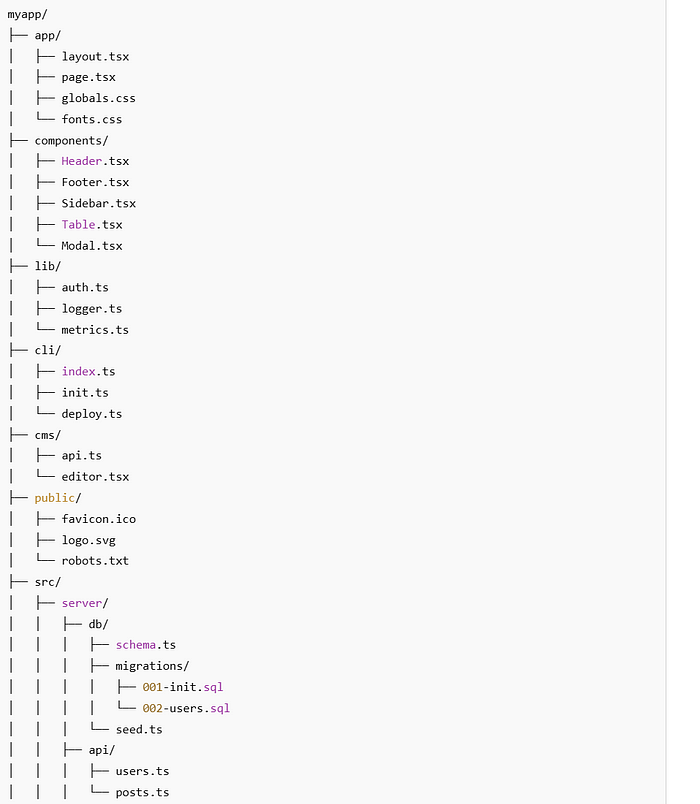
压缩后看起来像这样:
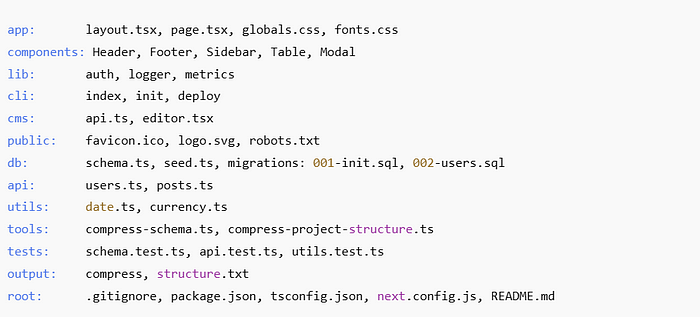
说实话,我更喜欢这种更简洁的布局,尽管当有深层嵌套时可能会有点困难。
以下是让你的脚本工作的步骤:
// compress-project-ultra.ts – 高效压缩文件结构
// 运行方式:pnpm project:ultracompress
import fs from 'fs';
import path from 'path';
import fg from 'fast-glob';
// 分组以合并为一行的目录
const groups = [
'app', 'components', 'lib', 'cli', 'cms', 'public', 'db', 'api', 'utils', 'tools', 'tests', 'output', 'src/server/db/migrations'
];
const groupLabels: Record<string, string> = {
'src/server/db': 'db',
'src/server/api': 'api',
'src/server/utils': 'utils',
'src/server/db/migrations': 'migrations',
};
const flattenPath = (file: string) => file.replace(/\\/g, '/');
const run = async () => {
const files = await fg(['**/*.*'], {
ignore: ['node_modules/**', '.git/**', '.next/**', 'dist/**'],
onlyFiles: true,
cwd: process.cwd(),
});
const grouped: Record<string, string[]> = {};
const rootFiles: string[] = [];
for (const rawFile of files) {
const file = flattenPath(rawFile);
const segments = file.split('/');
// 匹配已知分组或使用文件夹名称
const group = (() => {
if (file.startsWith('src/server/db/migrations/')) return 'migrations';
if (segments.length === 1) return 'root';
for (const base of Object.keys(groupLabels)) {
if (file.startsWith(base)) return groupLabels[base];
}
return segments[0];
})();
const name = path.basename(file).replace(/\.tsx?$|\.jsx?$|\.css$|\.json$/g, '');
if (group === 'root') rootFiles.push(name);
else {
grouped[group] ||= [];
grouped[group].push(name);
}
}
const lines: string[] = [];
const groupOrder = [...groups, ...Object.keys(grouped).filter(k => !groups.includes(k))];
for (const group of groupOrder) {
if (!grouped[group]) continue;
const items = grouped[group].sort().join(', ');
lines.push(`${group}: ${items}`);
}
if (rootFiles.length > 0) {
lines.push(`root: ${rootFiles.sort().join(', ')}`);
}
const output = lines.join('\n');
const outPath = path.resolve('output', 'structure-ultra.txt');
fs.mkdirSync(path.dirname(outPath), { recursive: true });
fs.writeFileSync(outPath, output);
console.log('✅ 极度压缩后的结构已保存至:', outPath);
};
run();
现在再次传递这些较小的文件作为上下文,我发现搜索文件的速度快了很多。
顺便说一下,在较大的项目中,我也发现可以轻松地将上述文件传递给 ChatGPT,并与压缩模式一起使用,这样它可以更快地了解你的项目,这是一个巨大的优势。
这些节省可能看起来微不足道,但请记住,Cursor 只有大约 20,000 个上下文令牌,所以我们需要尽可能压缩所有内容。
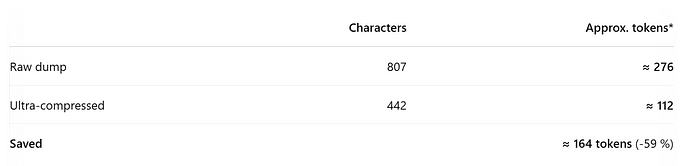
正常的查询通常大约是 100 到 200 个令牌长。
因此,通过采用这种策略,我们可以用文件目录和结构压缩 300 个令牌的上下文。记住我们需要精确性,每次模型调用和读取 200 行代码时,大约会消耗 1,500 到 2,000 个令牌。
4、结束语
我们正在为处理更大的项目奠定基础,这只是系列的第一部分。在许多领域我们还可以实现更多的优化。
这只是务实的良好开端,我真的很想介绍管道和工具的概念,帮助你更有效地提示。
我很好奇你在大型项目中有何其他技巧!
原文链接:Speeding up your Cursor AI project on larger codebases — Part 1: Compression
汇智网翻译整理,转载请标明出处
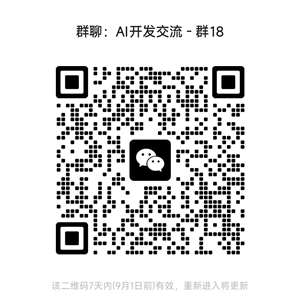